最終更新日:
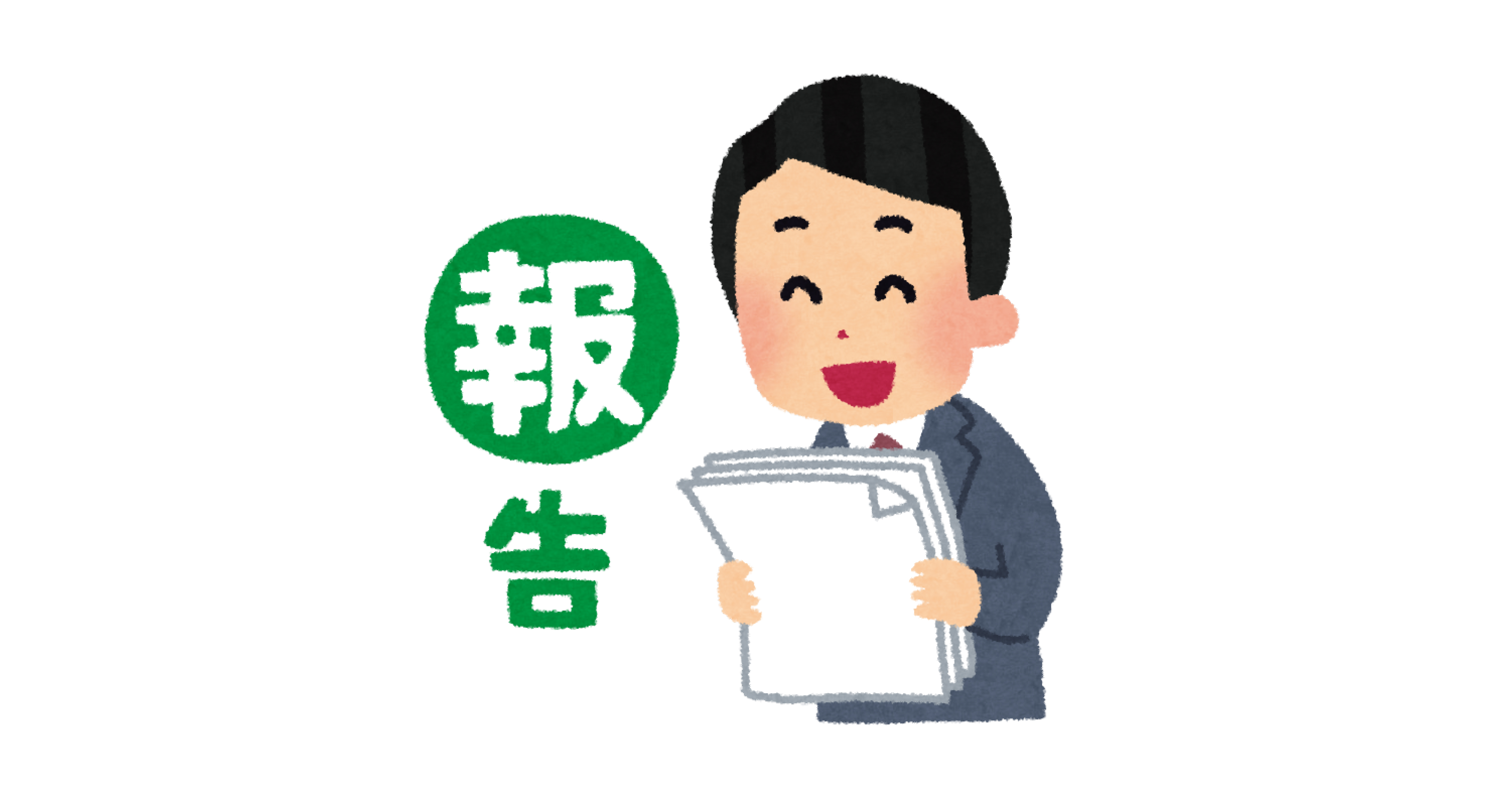
目次 [非表示]
Web空間上のテキスト・データからの事実収集・知見抽出
ウェブ空間には、1人の人間が読みきれない分量の文章データが蓄積されています。
ウェブ上の文書の山のなかには、どのような、事実(認定)に関する知見や、意見・主張(主義主張)や提案が書かれているのか。
さらに、ウェブ空間上には、膨大な量の静止画像と動画データがあります。
これらの(動)画像データについても、そこに収められている出来事に関する情報を、短い要約文の形で、内容を自動的に受け取ることができると、とても便利です。
テキスト・データと(動)画像データから、そこに記録されている「意味」を大づかみに(=一定の抽象度のレベルで)取り出して、短い要約文に置き換えてくれるアルゴリズムが、複数考案されています。
この記事では、こうしたニーズに応える技術の動向について、紹介します。
「事実の認定」と「情報の要約」には、特定の「観点」が伴う
ところで、ある文書(文章)を要約する際、複数の観点から要約文を作成することができます。
同じ文章(文章)を解析対象として取り上げていても、どのような観点からその文書を要約するかによって、異なる情報を含んだ要約文を生成することができます。
ここでいう「特定の観点」とは、特定の「トピック」を指す場合もあれば、特定の「視点」を含む場合もあります。
『特定の「視点」』という場合も、さまざまな「視点」が含まれます。
一例として、複数の当事者が関わる出来事の動静を伝える観測記事が解析対象の文書である場合を考えてみましょう。
その文書に登場する当事者のうち、「当事者A」の「視点」を一人称の「主語」に設定した上で、”Aさんから見て、ある期間にある事象がかくかくしかじかの成り行きで推移した”という要約文を作成することができます。
その要約文は、Aさんとは別の「Bさん」を一人称の「主語」に設定して作成された、”Bさんから見て、ある期間にある事象がかくかくしかじかの成り行きで推移した”という短文とは、そこに描かれている状況の捉え方(見え方・意味付け)が異なるはずです。
文書を読む速度と、文書を読むために使うことのできる時間の両方に制約のある人間に代わって、機械(AIモデル)が、ウェブ上の文書の山に、どのような事実(認定)や意見・主張や提案が書かれているのか、要約してくれる場合、どのような観点から文書(群)を要約してほしいのか、AIモデルは人間から指示を仰がねばなりません。
特定の観点に依拠した情報要約技術:Query focused abstract summarization(task)
人間から指示された「観点」に基づいて、AIモデルが文章要約を行うという課題(task)は、自然言語処理の研究の世界で、Query focused abstract summarization(task)と呼ばれています。
1は、北京大学とテンセント社(Tencent Inc.)の論文、2はFacebook AI Researchの論文です。
- Shen Gao et.al., Abstractive Text Summarization by Incorporating Reader Comment, AAAI’19
- Angela Fan, David Grangier & Michael Auli, Controllable Abstractive Summarization, the 2nd Workshop on Neural Machine Translation and Generation, 2018
- Tal Baumel, Raphael Cohen & Michael Elhadad, Topic Concentration in Query Focused Summarization Datasets, AAAI’16
テンセント社から提案されているShen Gao et.al.(2019)の手法では、元となる文章(以下のdocument)に対して、要約の主題(観点)が、人間からAIモデルに与えられるコメント(comments)として、AIモデル側に与えられています。
以下では、「コメント」として、“Toyota’s investment in Uber is a wise choice” 及び “$500 million investment is really a lot of money!” が与えられています。
そのため、「トヨタがウーバー社に対して行った投資額は、妥当な金額とみなすことができるか否か」という問いかけ(=主題)に応える要約文を、元の文書(document)から自動生成することが求められています。
上記の「問いかけ」に答えていない要約文は、不正解になります。
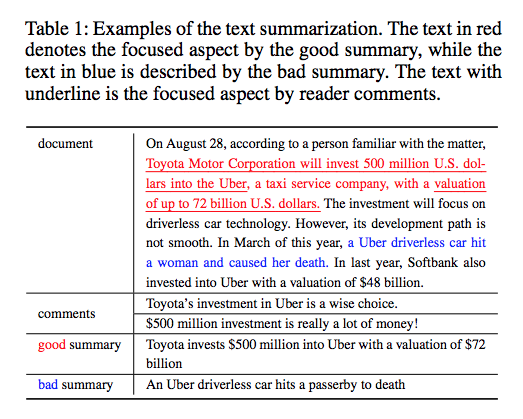
Tencent社ほかの論文1より転載。
なお、Seq2seqモデルなどを用いて、文書要約を行う過程で、要約元の文書に書かれている事実と異なる事実が記述された短文が、誤って生成されてしまう傾向があるとの研究もあります。
要約元の文書に盛り込まれている「事実認定」を損なわい要約文を自動生成を行うことを担保したアルゴリズムとしては、以下が提案されています。
香港理工大学(The Hong Kong Polytechnic University)と北京にあるMicfosoft Research研究所から出ている論文です。
なお、要約元の文書に記述されている「事実認定」が客観的に妥当かどうかは、上記の論文で提案されているモデルとは別の判定器を追加的に用いて、判断する必要があります。
参照先の元文書から「どのような情報」を要約したいのかの指示を、質問文の形で、人間から受け取るモデルも提案されています。
- Tal Baumel, Matan Eyal & Michael Elhadad, Query Focused Abstractive Summarization: Incorporating Query Relevance, Multi-Document Coverage, and Summary Length Constraints into seq2seq Models, Arxiv 2018.
- Johan Hasselqvist, Niklas Helmertz & Mikael Kågebäck, Query-Based Abstractive Summarization Using Neural Network, Arxiv 2017
- 西野 正彬ほか「クエリ中の語を含むことを保証するクエリフォーカス要約」, 言語処理学会第18会年次大会, 2012年3月
どのような観点から要約文を作成するかをAIモデルの判断に委ねるモデル
どのような観点から要約文を作成するかをAIモデルの判断に委ねるモデルは、伝統的なAbstract summarization taskになります。
Inspired by how humans summarize long documents, we propose an accurate and fast summarization model that first selects salient sentences and then rewrites them abstractively (i.e., compresses and paraphrases) to generate a concise overall summary. W
(関連)画像要約文・動画要約文生成における要約観点の指定
なお、複数の物体が写り込んでいる静止画像や動画をもとに、(動)画像に捉えられた状況を説明する文章を生成する際にも、(動)画像内の「どの物体」に着目するのかに応じて、複数の状況説明文を用意することができます。
人間の側から、「どの物体」に着目するのかをAIモデル側に指示を出してあげることで、人間に指定された物体を「主題」にした状況説明文を生成することができるモデルが提案されています。
以下のモデルでは、人間が、(動)画像中の任意の1つの物体を意味する単語(名詞)をAIモデルに与える形で、作成すべき状況説明文の「主題」が何であるのかをAIモデルに指示出しを行います。
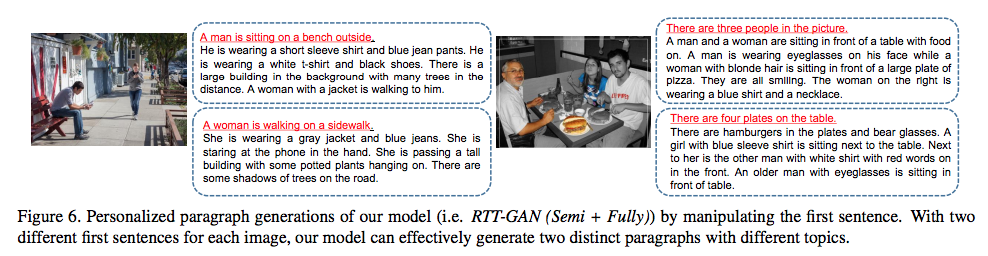
Xiaodan Liang et.al., Recurrent Topic-Transition GAN for Visual Paragraph Generation, Arxiv 2017より転載。
Generating a full paragraph description for an image/video is challenging. First, paragraph descriptions tend to be diverse, just like different individuals can tell stories from personalized perspectives. As illustrated in Figure 1, users may describe the image starting from different viewpoints and objects. Existing methods [16, 35, 19] deterministically optimizing over single annotated paragraph thus suffer from losing massive information expressed in the image. It is desirable to enable diverse generation through simple manipulations.
( 中略 )
Our model allows diverse descriptions from a single image by manipulating the first sentence which guides the topic of the whole paragraph. Semi-supervised learning is enabled in the sense that only single-sentence caption annotation is required for model training, while the linguistic knowledge for constructing long paragraphs is transfered from standalone text paragraphs without paired images.
この論文で提案されている手法は、GANモデルのフレームワークを採用しています。
Inspired by Generative Adversarial Networks (GANs) [6], we establish an adversarial training mechanism between a structured paragraph generator and multi-level paragraph discriminators, where the discriminators learn to distinguish between real and synthesized paragraphs while the generator aims to fool the discriminators by generating diverse and realistic paragraphs. The paragraph generator is built upon dense semantic regions of the image, and selectively attends over the regional content details to construct meaningful and coherent paragraphs. To enable long-term visual and language reasoning spanning multiple sentences, the generator recurrently maintains context states of different granularities, ranging from paragraph to sentences and words. Conditioned on current state, a spatial visual attention mechanism selectively incorporates visual cues of local semantic regions to manifest a topic vector for next sentence, and a language attention mechanism incorporates linguistic information of regional phrases to generate precise text descriptions. We pair the generator with rival discriminators which assess synthesized paragraphs in terms of plausibility at sentence level as well as topic-transition coherence at paragraph level.
(動)画像中の1つの物体を主題に据える状況説明文を、物体の数だけ文生成する手法
(動)画像中の1つの物体を主題に据える状況説明文を、物体の数だけ文生成します。
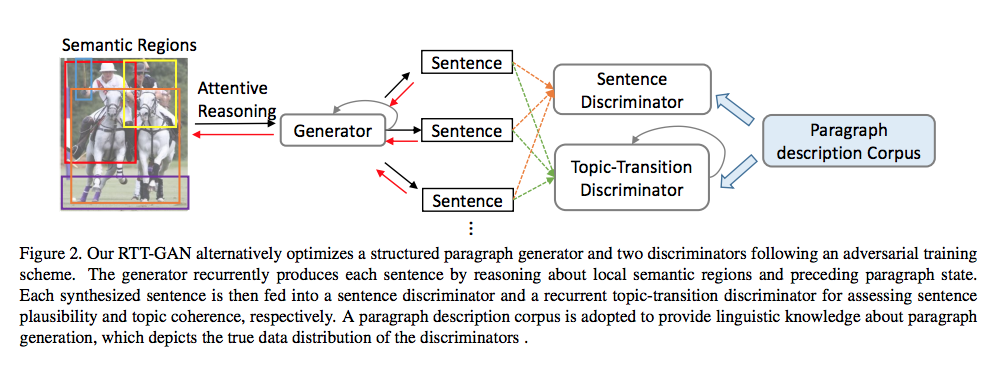
Xiaodan Liang et.al., Recurrent Topic-Transition GAN for Visual Paragraph Generation, Arxiv 2017より転載。
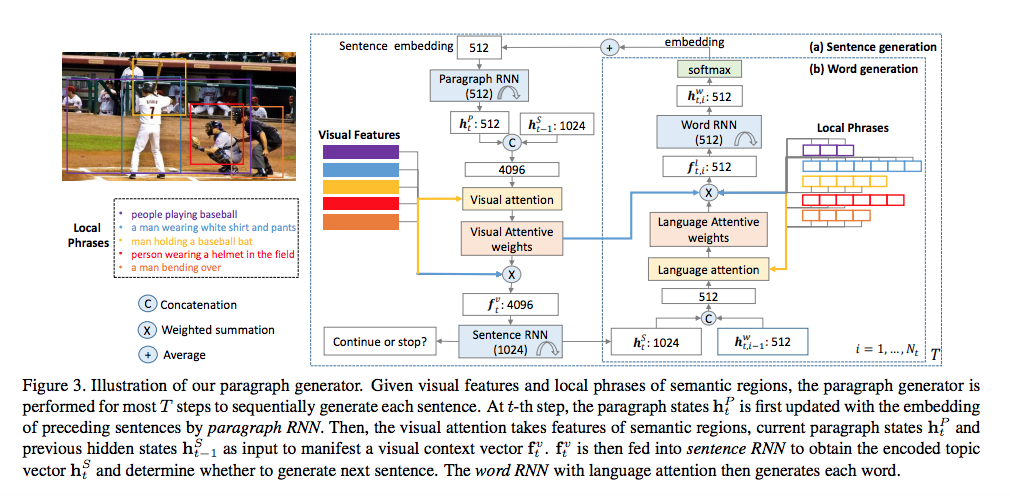
Xiaodan Liang et.al., Recurrent Topic-Transition GAN for Visual Paragraph Generation, Arxiv 2017より転載。
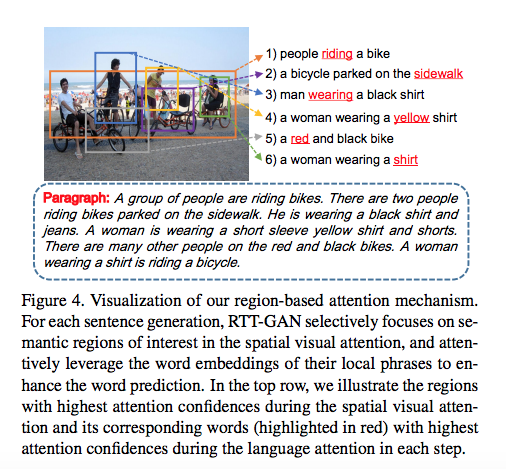
Xiaodan Liang et.al., Recurrent Topic-Transition GAN for Visual Paragraph Generation, Arxiv 2017より転載。
なお、(動)画像の任意の部分領域を着目すべき主題の領域に定めた上で、(動)画像全体についての状況説明文を生成する手法は、以下の論文で提案されています。
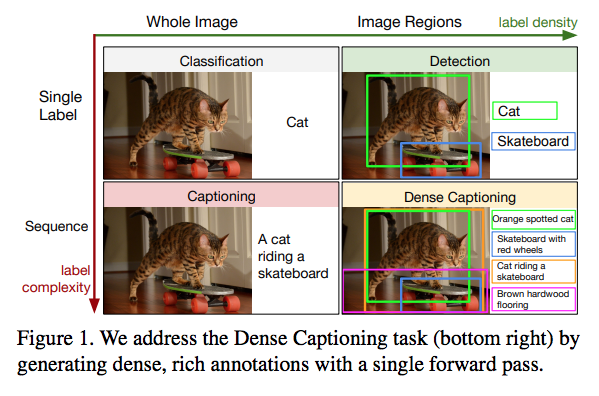
Justin Johnson, Andrej Karpathy & Li Fei-Fei, DenseCap: Fully Convolutional Localization Networks for Dense Captionin, Arxiv 2015より転載。
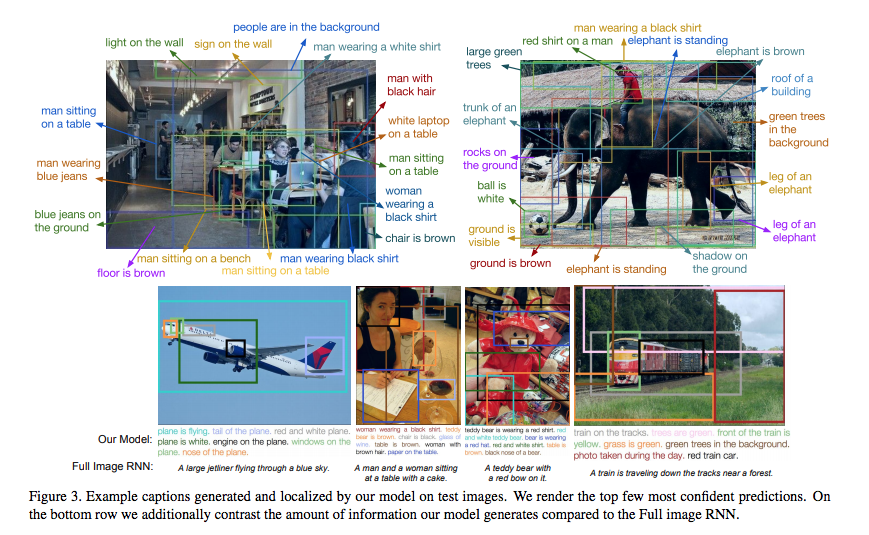
Justin Johnson, Andrej Karpathy & Li Fei-Fei, DenseCap: Fully Convolutional Localization Networks for Dense Captionin, Arxiv 2015より転載。
以下のアーキテクチャによって、実現されています。
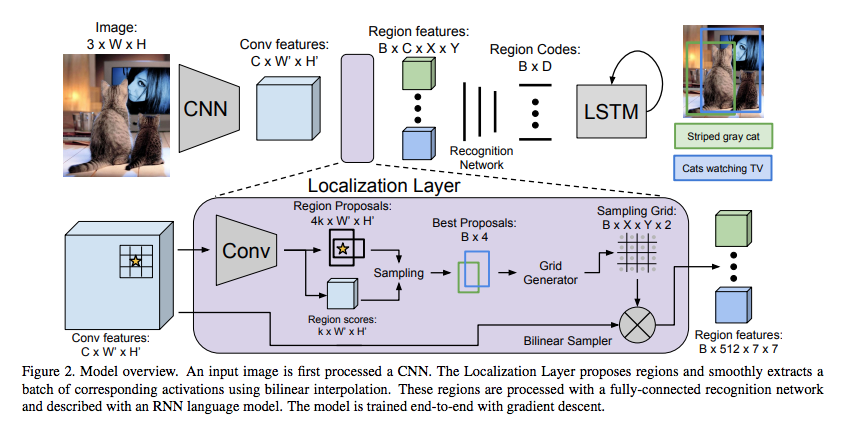
Justin Johnson, Andrej Karpathy & Li Fei-Fei, DenseCap: Fully Convolutional Localization Networks for Dense Captionin, Arxiv 2015より転載。
(発展的考察)画像中の極小領域を高精度に画像解析しうるモデルとの組み合わせ
今日の画像解析器は、画像に写り込んだきわめて小さなサイズの物体についても、画像を鮮明化し、物体識別や人物同定を行う能力を獲得しつつあります。
中国科学院(Chinese Academy of Sciences)他から公開された以下の論文は、人間の群衆の様子を捉えた一枚の画像(wild crowd。論文中では、“wild”と記述)のなかに映り込んでいるすべての人間の表情画像を拡大し、鮮明化させる手法が提案されています。
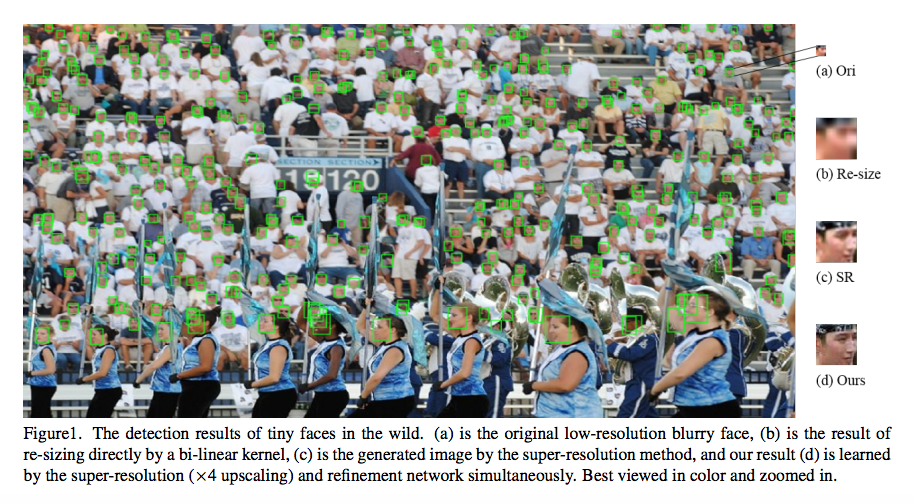
Yancheng Bai, et.al., Finding Tiny Faces in the Wild with Generative Adversarial Networkより転載。
こうした技術と組み合わさることで、人間である解析者が、(動)画像中に映り込んだ任意の(サイズの大小にかかわらず)事物にフォーカス(attention)を当てて、(動)画像に映り込んでいる状況を英文や中国語文や日本語の文の形に要約させて、空間動態に関するモニタリング(要約)情報を受け取ることができるようになりつつある可能性が高いと認識するべきなのかもしれません。
(Yancheng Bai, et.al.論文で提案されている手法)
GANモデル(敵対的生成モデル)の枠組みを用いることで、極小サイズ且つ低解像度の画像を元に、サイズを大きく拡大し、さらに解像度が高精細化された画像を生成する画像処理モデルを提案しています。
Abstract
Face detection techniques have been developed for decades, and one of remaining open challenges is detecting small faces in unconstrained conditions. The reason is that tiny faces are often lacking detailed information and blurring. In this paper, we proposed an algorithm to directly generate a clear high-resolution face from a blurry small one by adopting a generative adversarial network (GAN). Toward this end, the basic GAN formulation achieves it by super-resolving and refining sequentially (e.g. SR-GAN and cycle-GAN)
提案モデルの入出力関係は、以下の通りです。
入出力関係
- 入力データ:人物の群衆画像データ(10-30pixelサイズの極小の人物表情画像と、看板や背景画像など、Non-faces画像が混在した画像データ。WIDER FACE datasetのHardカテゴリ画像を採用)”the original low-resolution blurry face”
- 出力データ:極小サイズ(10-30pixel)の人物画像を拡大(“up-sampling”)・鮮明化(“refining”)させた人物識別可能な画像 “a clear high-resolution face”
Extensive experiments on the challenging dataset WIDER FACE demonstrate the effectiveness of our proposed method in restoring a clear high-resolution face from a blurry small one, and show that the detection performance outperforms other state-of-the-art methods.
データ処理過程
- 極小画像の拡大処理(“up-sampling”)
- 拡大した画像の鮮明化処理(“refining”):(ぼやけ(“blurry”)・照り(“illumination”)の除去と、非正面の姿勢(“arbitrary poses”)の正面化などを含む処理。
このような、画像の拡大と高精細化(鮮明化)という2段階の処理が施されることで、入力画像(a)は、最終的に(d)の画像に加工されて、モデルから出力されます。
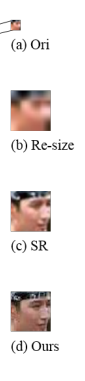
この様子を論文中の別の画像例で説明してみます。
上段(Original)の画像が、提案モデルに入力された元画像です。(但し、実際のサイズは小さすぎて視認しずらいため、下段の拡大された後の画像と同じサイズに修正した上で掲載されている)
そして、下段(Ours)の画像が、対応する上段の画像に拡大処理+鮮明(高精細)化処理が施された後の画像です。この下段の画像が、モデルから出力(生成)される画像です。
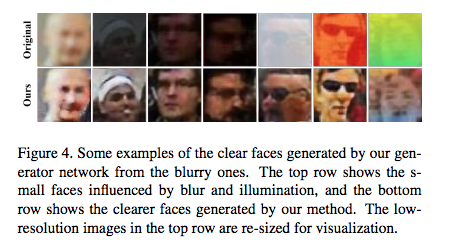
これまでも、画像を高精細化(super-resolution)させるGANは、SR-GANとして提案されてきましたが、生成される画像は、ぼやけた(blurry)画像でした。
提案モデルは、上記の下段の画像を生成できるため、これまでのモデルよりも優れたモデルであるという主張がなされています。
Recently, GAN has been applied to super-resolution (SRGAN) [17] and has obtained promising results.
Compared to superresolution on natural images, face images in the wild are of arbitrary poses, illumination and blur, so super-resolution on face images is much more challenging.
More importantly, the high resolution images generated by SRGAN are blurry and lack fine details especially for low-resolution faces, which are unfriendly for the face classifier
モデルのネットワーク構成
- 極小画像の拡大処理を担う“up-sampling sub-network”
The up-sampling sub-network first up-samples a lowresolution image and outputs a 4× super-resolution image, and this super-resolution image is blurring when the small faces are far from the cameras or under fast motion.
2. 拡大した画像の鮮明化処理を担う“refinement sub-network”
Then, the refinement sub-network processes the blurring image, and outputs a clear super-resolution image, which is easier for the discriminator to classify the faces vs. non-faces.
なお、2.で取り除かれる画像の「ぼやけ」が発生する要因としては、上記の1を記述した文のくだりで、画像を撮影したカメラから遠距離に位置する人物や、高速で移動中(“under fast motion”)の人物を撮影した場合が、例示されています。
this super-resolution image is blurring when the small faces are far from the cameras or under fast motion.
モデル概要:GANモデルを拡張したもの
GAN(敵対的生成モデル:Generative Adversary Network)モデルを拡張したモデルを採用しています。
- GANのGenerator networkのsub network (1): “Up-sample sub-network“:10-30pixelの人物表情画像を画像拡大する(Up-sampling処理)
- GANのGenerator networkのsub network (2): “Refinement sub-network“:拡大した画像から、ぼやけ(blurry)や照り(illumination)を除去し、姿勢を正面を向いた方向に修正する(Refinement処理)
- GANのDiscriminator network:G-subnetwork(2)から出力された生成画像が、(1) 人物の氷像画像であるのか否か(“Face” or “Non-face”) 及び (2) 本物の顔画像か、合成された偽物の顔画像か(“fake” or “real”, HR(the natural (high-resolution) image) or SR “generated super-resolution image”)の2つを判定する。
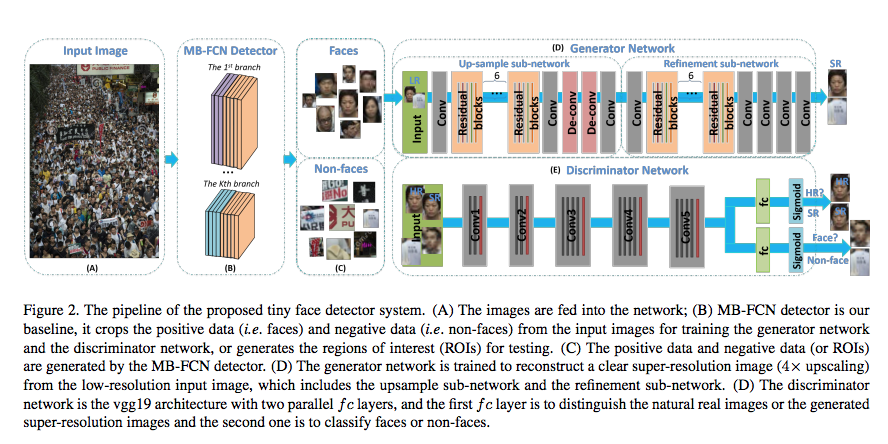
なお、入力データをGANモデルの2本の柱であるGenerator部とDiscriminator部に入力する前に、入力データである群衆画像を構成する個々の部分画像領域が、「人物の顔画像が写り込んだ画像(Faces)」であるのか、「人物の顔画像が映りこんでいない画像(Non-faces)」であるのかを判別する分類器であるMB-FCN Detector が、間に挟み込まれています。
Our baseline MB-FCN detector is based on ResNet50 network [9], which is pre-trained on ImageNet. All hyperparameters of the MB-FCN detector are the same as [1].
For training our generator and discriminator network, we crop face samples and non-face samples from WIDER FACE [31] training set with our baseline detector.
上記の記述にあるように、このMB-FCN Detector(顔画像判定器)は、以下の論文で提案されているモデルを借用しています。
GANモデルの拡張ポイント(3点)
提案モデルは、従来のGANモデルを以下の3点で「拡張」したものです。
(1)Generator部を2つのsub-networkに分けた。(画像の拡張処理部および鮮明化処理部)
(2)Discriminator部を2つの「FC(全結合)層+Sigmoid層」に分けた。(「人物の表情画像か否か」を判定するFC層+Sigmoid層と、「大きく(且つ)鮮明に撮影された画像か、それとも、極小画像を拡大化処理&鮮明化処理を通して得られた画像か」を判定するFC層+Sigmoid層)
(3)損失関数を、3つの損失関数で構成される(1つの)統合損失関数として設計した。
GANモデルの損失関数は、以下の3つの損失関数を最終的に1本の定義式(損失関数式)にまとめたものになります。
3つの損失関数は、(1)Pixel-wise loss. Lmse(w), (2)Adversarial loss. L_{adv} そして (3)Classification loss. L_{clc} です。
- Pixel-wise loss. Lmse(w) : モデルへの入力画像である「小さく、ぼやけた画像」と、モデルが生成した「高精細な画像」を見分ける判別精度を計量計量評価する損失関数。以下の定義式で表現されているように、後者の『モデルが生成した「高精細な画像」』は、まず最初に、I^{LR}_{i}:「小さく、ぼやけた画像」をGenerator1の処理関数(G1_{w1})に投入して画像サイズを拡大させた後に、さらに、Generator2の処理関数(G2_{w2})に投入して画像を鮮明化させた結果として、得られた画像として定義されています。
[期待される役割]
モデルから出力される(拡大処理と鮮明化処理を施された)画像が、「撮影当初から大きくて、鮮明であった画像」(the super-resolution ground truth)に(極限まで)近づくように、モデル全体を制御する役割が期待されています。
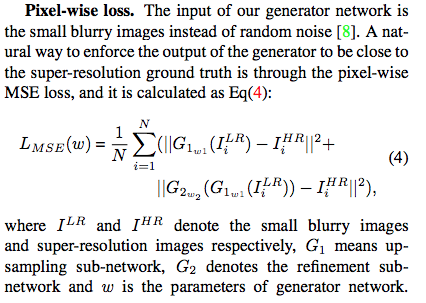
2. Adversarial loss. L_{adv} : 生成された画像が、「撮影当初から高精細(natural super-resolution)であった、本物の画像」であるのか、「撮影当初は不鮮明であった画像を人為的に高精細化処理を施した画像」であるのかの判別精度を計量評価する損失関数
In Eq(5), the Dθ(Gw(I LR i )) is the probability that the reconstruction image Gw(I LR i ) is a natural super-resolution image.
後者の「撮影当初は不鮮明であった画像を人為的に高精細化処理を施した画像」は、「撮影当初は不鮮明であった画像」を表すI^{LR}_{i}(LRは、Low-resolutionを意味する)を、人為的な画像高精細化処理関数であるG_{w}に投入することで得られます。
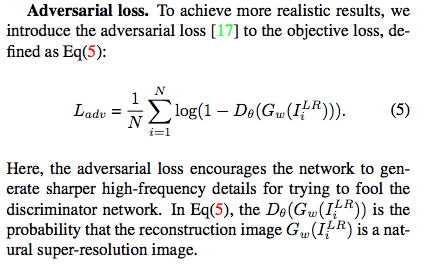
3. Classification loss. L_{clc} : 「ある高精細な画像」(「撮影当初から高精細であった本物の画像」[I^{HR}_{i}: the natural real high resolution images, the high-resolution real natural images)なのか、それとも、「撮影当初は不鮮明であった画像」(I^{LR}_{i}: the small blurry images) に対して、「人為的な高精細化処理」(関数G_{w})を施した「画像」なのか、の別は問わない)が、「人物の顔画像であるか否か」の判別(classify)精度を計量評価する損失関数。
[期待される役割]
提案モデルから最終的に生成される画像が、人物の表情画像か、非ヒト表情画像なのかを判別しやすい画像になるように、モデルに制御をかける役割が期待されている損失関数です。
Our classification loss plays two roles, where the first is to distinguish whether the high-resolution images, including both the generated and the natural real high-resolution images, are faces or non-faces in the discriminator network.
The other role is to promote the generator network to reconstruct sharper images.
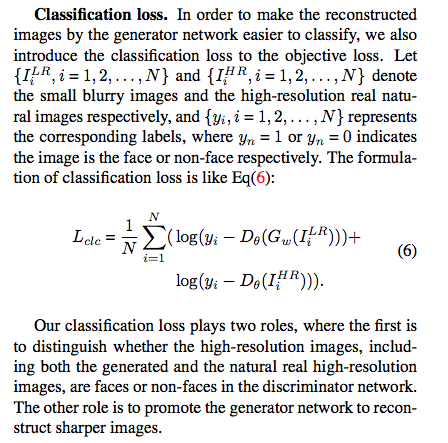
上記の3つの損失関数を1本の損失関数に束ねたものは、以下になります。モデルの学習時に用いられる損失関数は、この損失関数です。
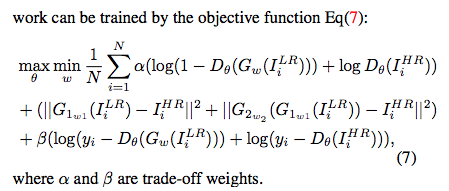