最終更新日:
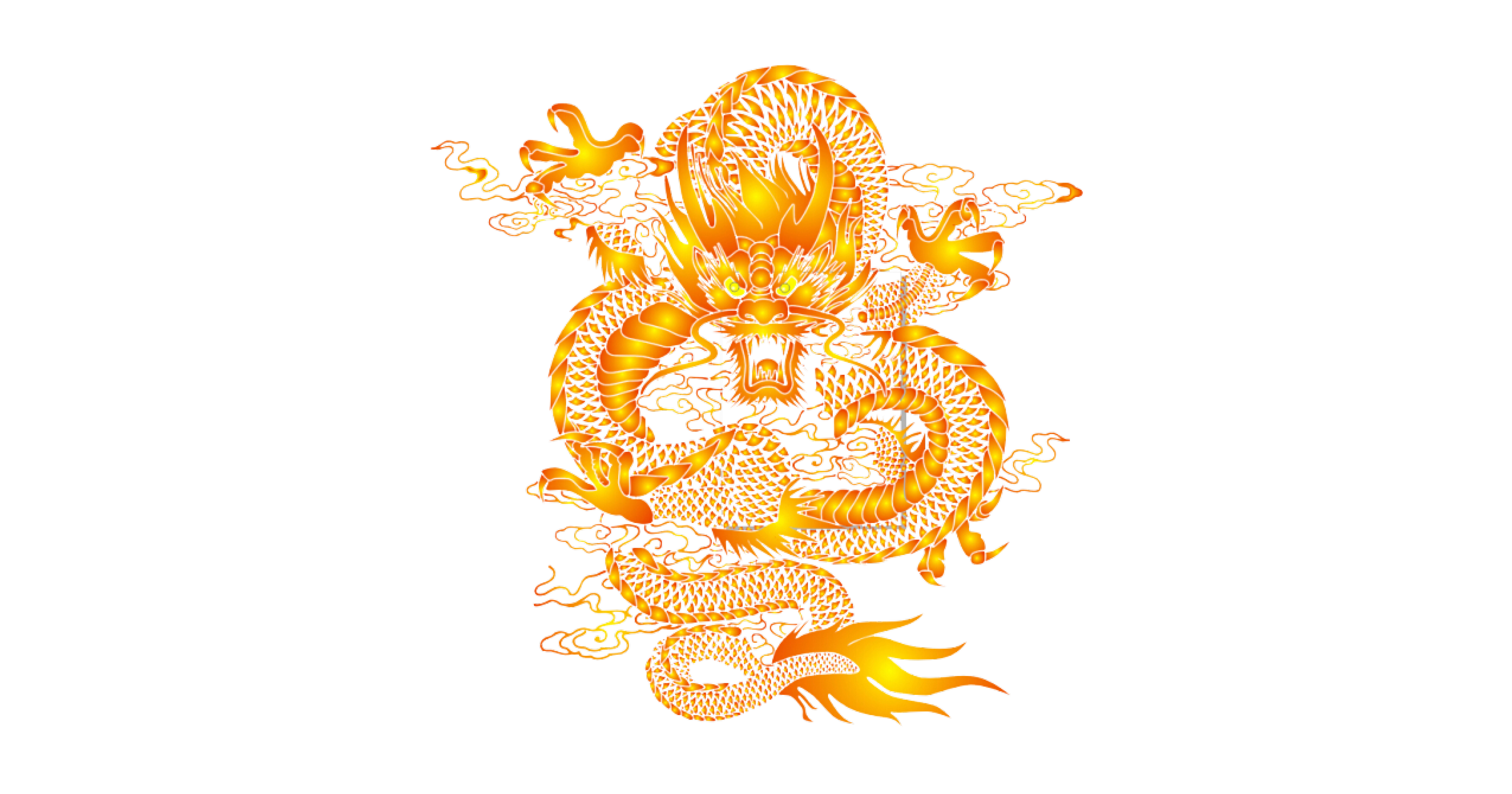
中国の都市部では、日用品の買い物で代金を支払うシーンや、タクシー配車アプリで手配した乗用車の運賃支払い場面で、顔認識による支払い決済が、すでにあたりまえの光景になっています。
クレジット・カードで支払いをすると、財布からカードを取り出さなければならない上に、カード読み取り機がクレジット会社の通信センターと通信するのに数秒かかり、おまけに肉筆で署名までしなくてはならなかったりと、とても不便です。
センサーに顔をかざすだけで瞬間的に代金支払いが完了してしまう光景は、紙幣や硬貨による現金支払い率が高止まりしている東京に暮らす私達にとっては、なんとも羨ましい光景です。(そのかわり、自分がどこで、だれと、何を購入したのかは、事業者や政府に知られてしまうのが、監視社会と言われる中国の現状です)
また、中国の都市部では、市街地や道路交通網に張り巡らされたAI搭載型の「インテリジェント監視カメラ」を用いて、犯罪者や犯罪予備群の動向に目を光らせることで、すでに発生した犯罪を捜査したり、今後起こりうるテロ犯罪を思いとどまらせる犯罪抑止(防遏(ぼうあつ))に用いているとされています。
このように、中国では、社会インフラに対する画像解析のAIシステムの導入が進んでいると報じられています。
この連載シリーズでは、中国の研究機関・企業から公開されている(動)画像解析領域の論文を、企業別・研究機関別に取り上げて論じていきます。
「連載第一弾」の本記事の冒頭では、中国発の(動)画像解析テクノロジー企業として、研究者や投資家から注目を集めているセンスタイム社(SenseTime Group, 商湯科技)とメグビー・テクノロジー社(Megvii Technology Inc.,北京曠視科技)を取り上げます。また、3社目の会社として、WATRIX社(「银河水滴科技」社)を取り上げます。
1社目のセンスタイム社は、自動車や歩行者など、移動中の物体をリアルタイムで車両識別・人物識別する移動体認識領域での技術の高さが注目されている企業です。
また、ZDNet Japan (2018/11/08 07時00分)「顔認識が強みの中国AIベンチャー、センスタイム–ホンダと自動運転の共同開発も」でのインタビューのなかで、「SenseTimeの副社長で、日本法人のセンスタイムジャパンでCEOを務める勞世竑氏」は、「SenseTimeの顔認識技術は年齢変化や照明変化に強みがある。中国の身分証は有効期間が20年と長いため、加齢による顔の変化に対応できる技術が必要になる。また、室内・屋外で照明環境が異なっても正しく認識できる」と述べています。
センスタイム社をめぐっては、日本のホンダ自動車やDeNA社が、同社との提携を発表しています。
- [プレス・リリース] HONDA News Release (2017年12月07日)「中国のSenseTime社と、自動運転のAI技術に関する共同研究開発契約を締結」
- [プレス・リリース] :DeNA (2019年01月22日)「DeNAとSenseTimeが業務提携 SenseME、SenseMedia等のAIソリューションの日本国内での販売を開始」
2社目のメグビー・テクノロジー社は、人間の顔認識サービスを展開している会社です。従来の「顔認識」技術に、「ジェスチャー認識」(Gesture Recognition)と「目線の認識」(Gaze Estimation)の2つの技術を組み合わせることで、任意の方角を向いた顔画像であっても、被写体が誰であるのかを高い精度で判別しうる技量の高さに、評価が集まっているようです。このあたりについては、本記事で後にとりあげるCOURRIER Japan誌が伝えています。
また同社は、同社から出ている以下の論文のなかで取り組まれているように、人間の顔の幾何学的な形状を、Homographyなどの幾何学の知見を用いた手法で解析を行うことで、さまざまな方向を向いた人物の表情画像から、その人物が誰であるのかを精度よく識別することができる技術を蓄積しているようです。
Abstract. In this paper, we propose a method, called GridFace, to reduce facial geometric variations and improve the recognition performance. Our method rectifies the face by local homography transformations, which are estimated by a face rectification network. To encourage the image generation with canonical views, we apply a regularization based on the natural face distribution. We learn the rectification network and recognition network in an end-to-end manner. Extensive experiments show our method greatly reduces geometric variations, and gains significant improvements in unconstrained face recognition scenarios.
3社目に紹介するWATRIX社(「银河水滴科技」社)は、日本ではまだほとんど知られていない会社ですが、COURRIER Japan誌によると、『歩き方やシルエットから個人を特定できる歩行認識技術「Watrix」』(COURRIER誌)で注目されているようです。この歩き方から個人を特定する技術は、日本語では「歩様・歩容(ほよう)」認識という言葉があるようです。(参考1・参考2)
COURRIER誌によると、『同社の黄永禎・共同CEO(最高経営責任者)によると、カメラの50メートル先を歩く人間の特定が94%の精度で可能で、歩幅、歩長、歩調、スピード、両足の角度、尻の形状といったさまざまな動作を分析し、監視カメラの映像と照合。対象者が背を向けたり顔を隠したりしてもごまかせないため、黄CEOは「顔認証システムの盲点を埋める」と強調した』(以上、COURRIER誌記事)とのことです。
WATRIX社(「银河水滴科技」社)は、歩き方から人物を特定する技術の領域(市場)で、極めて高い技術力をもった会社として、いまから注目をしておくべきではないでしょうか。
この記事では、上記の3社を紹介したあと、本連載シリーズで取り上げる最初の論文として、中国科学院ほかから公開された論文であるYancheng Bai, et.al., Finding Tiny Faces in the Wild with Generative Adversarial Networkに着目して、その内容を見ていきます。
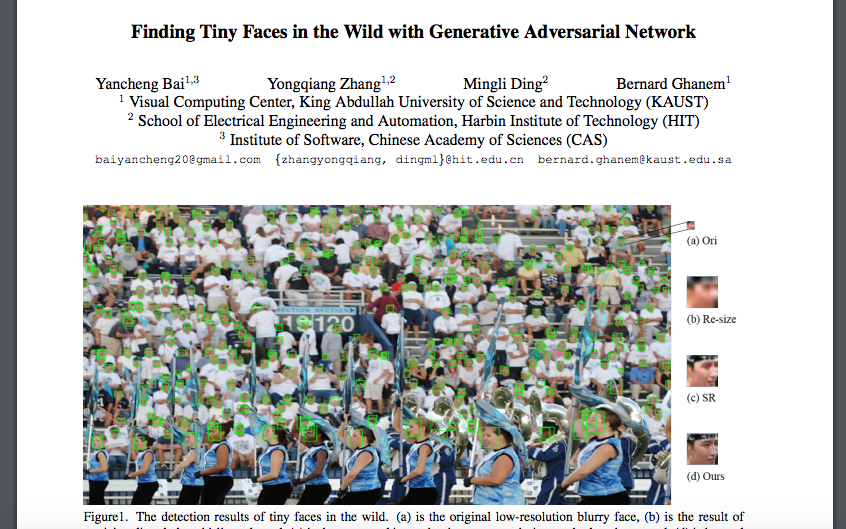
Yancheng Bai, et.al., Finding Tiny Faces in the Wild with Generative Adversarial Network
この記事の2回目以降の続編記事では、今回取り上げた中国ベンチャー2社から出ている英語論文の内容に詳しく踏み込んで見ていきたいと思います。
目次 [非表示]
発展著しい中国の(動)画像解析技術
中国の大学・研究機関および企業における(動)画像解析エンジンの研究開発のレベルには、目を見張るものがあります。
(動)画像解析の領域で注目されている中国企業としては、まずはセンスタイム社があります。
【 注目企業1】センスタイム社(SenseTime Group, 商湯科技)
Wikipedia(日本語版)「商湯科技」によると、センスタイム社は、2014年10月に香港中文大学情報工学科教授の湯 暁鴎(Tang Xiaoou)氏によって設立された会社であり、CEO(最高経営責任者)は共同創設者の徐 立(Xu Li)氏が務めているようです。
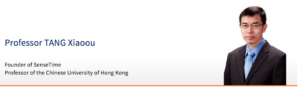
(転載元)https://www.hkstp.org/en/about-us/the-board/board-of-directors/members/prof-xiaoou-tang/
Google scholarによると、2019年2月4日現在、h-indexは101で、「10回以上引用された論文の本数」を表すi10-indexは、346のようです。2014年以降に公開した論文だけを集計対象に選んだ場合も、h-indexが82, i10-indexが270です。
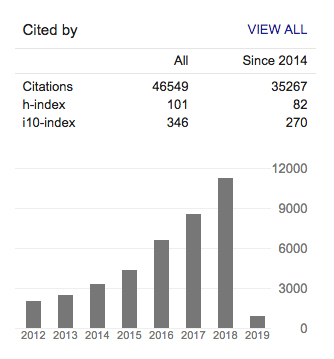
Google Scholar 2019年2月4日現在 https://scholar.google.com/citations?user=qpBtpGsAAAAJ&hl=en
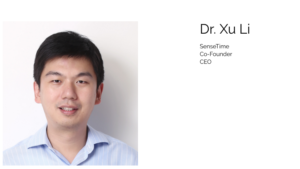
Kong X-Tech Startup Platformより転載 (http://www.hkxtech.com/dr-li-xu/?lang=en)
同じくGoogle scholarを見てみると、(2019年2月4日現在)h-indexは32で、i10-indexは、44のようです。2014年以降に公開した論文のみに絞ると、h-indexが30, i10-indexが41です。
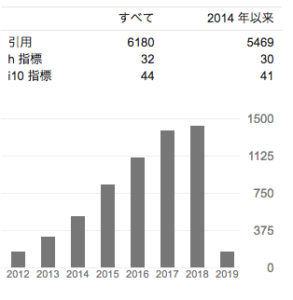
Google Scholar 2019年2月4日現在 https://scholar.google.com/citations?user=Go9TaC4AAAAJ&hl=ja
この会社の日本法人(センスタイム・ジャパン)は、京都府に居を構えており、日本で開催された「AI・人工知能Expo展」にも、大きなブースを構えて、画像認識エンジンの展示を行っていました。
儂もその展示会でセンスタイム・ジャパン社の展示を直接見たのですが、多量の移動物体(車や人)をリアルタイムに同時に物体検出して、車両同定と人物同定までを行うことができるデモ画面は、圧巻でした。
以下は、同社のSenseVideo-Aと名付けられたサービスで、車両や歩行者が誰かをリアルタイムに識別することができるサービスです。
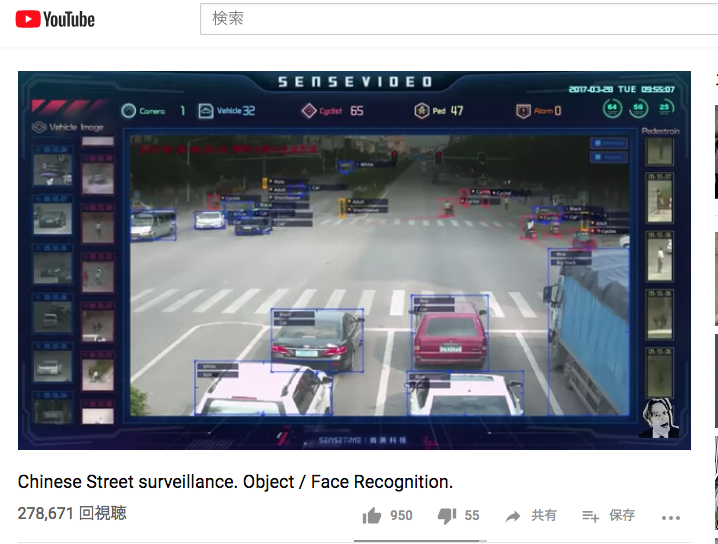
Robert McGregor (2017/09/22 に公開) Chinese Street surveillance. Object / Face Recognition.
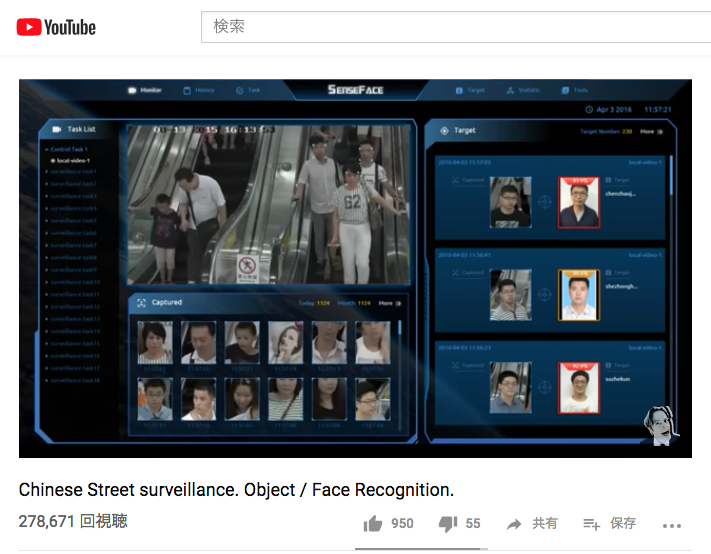
Robert McGregor (2017/09/22 に公開) Chinese Street surveillance. Object / Face Recognition.
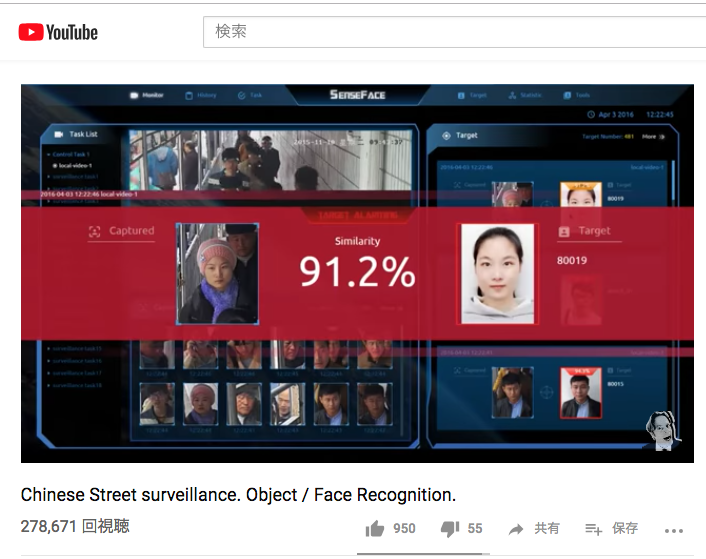
Robert McGregor (2017/09/22 に公開) Chinese Street surveillance. Object / Face Recognition.
センスタイム社(中国本社)のウェブページで開設されているSenseVideo-Aのページは以下になります。
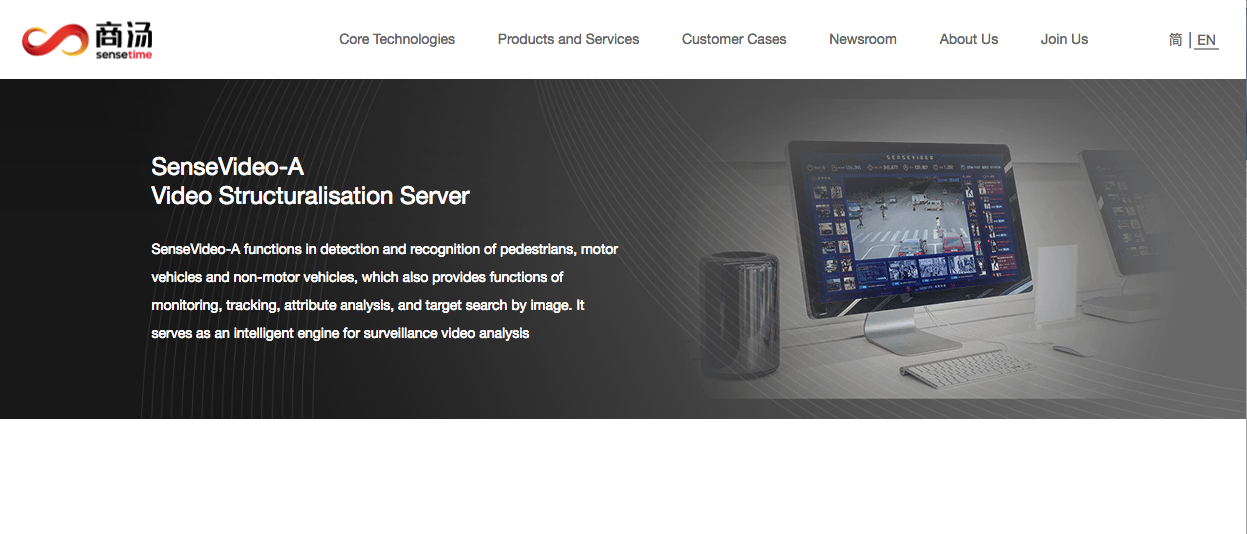
https://www.sensetime.com/intelligentVideo/86
センスタイム本社(中国)のウェブページはこちらです。
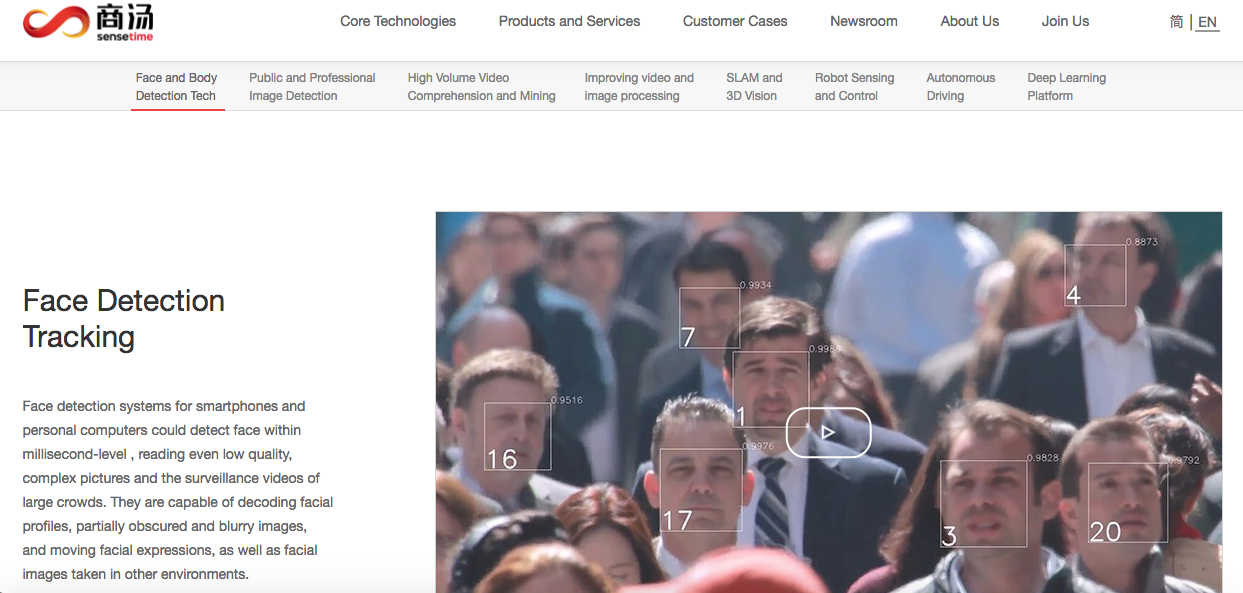
https://www.sensetime.com/core
以下がセンスタイム・ジャパンのウェブページです。
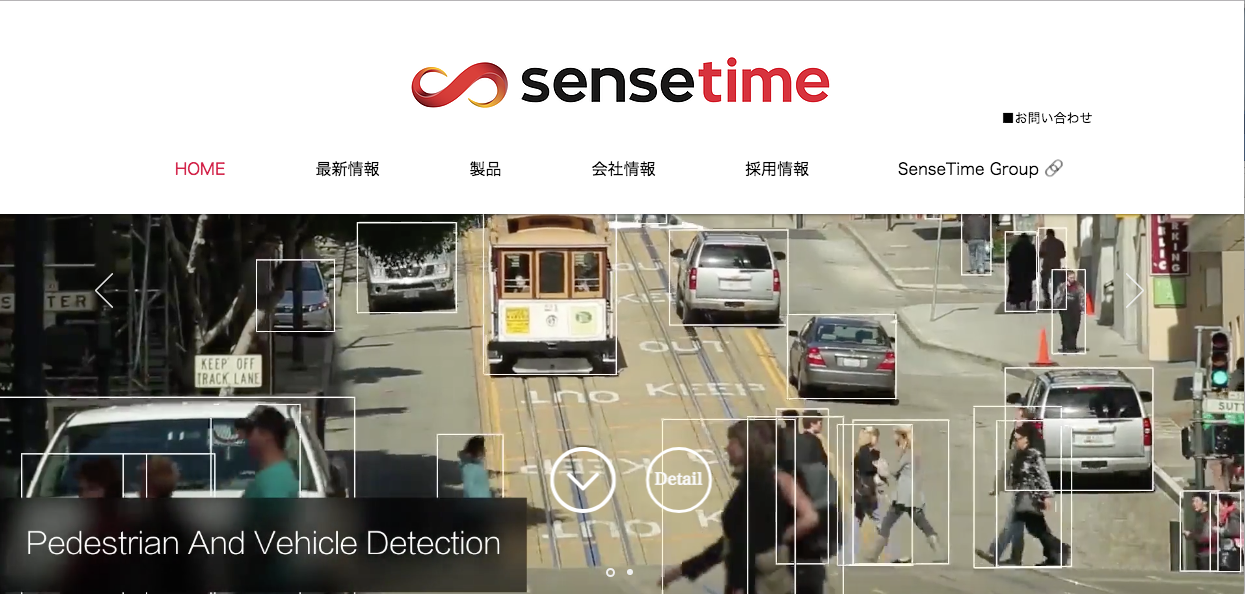
https://www.sensetime.jp/
センスタイム社から公開されている論文(一部)
この会社は、多くの論文が公開されています。
- Yi Wei et.al., Quantization Mimic: Towards Very Tiny CNN for Object Detection, Arxiv 2018
- Wayne Wu et.al., Look at Boundary: A Boundary-Aware Face Alignment Algorithm, Arxiv 2018
- Lu Sheng et.al., Avatar-Net: Multi-scale Zero-shot Style Transfer by Feature Decoration, Arxiv 2018
- Xuebo Liu et.al., FOTS: Fast Oriented Text Spotting with a Unified Network, Arxiv 2018
- Kaidi Cao et.al., Pose-Robust Face Recognition via Deep Residual Equivariant Mapping, Arxiv 2018
- Zhaoyang Zhang et.al., Temporal Sequence Distillation: Towards Few-Frame Action Recognition in Videos, ACM’18
- Zhao Zhong et.al., Practical Block-wise Neural Network Architecture Generation, Arxiv 2018
- Xiaoyang Guo et.al., Learning Monocular Depth by Distilling Cross-domain Stereo Networks, Arxiv 2018
- Jimmy Ren et.al., Accurate Single Stage Detector Using Recurrent Rolling Convolution, Arxiv 2017
- Dapeng Chen et.al., Improving Deep Visual Representation for Person Re-identification by Global and Local Image-language Association, ECCV’18
この論文では、画像に映っている人物が誰なのか識別(同定)するために、画像以外の補助情報(auxiliary information)として、画像のキャプション文を人物識別モデルに入力するアプローチが提案されています。
なお、この論文は、画像認識の分野で世界最高峰の学会であるECCVの査読を通過しています。
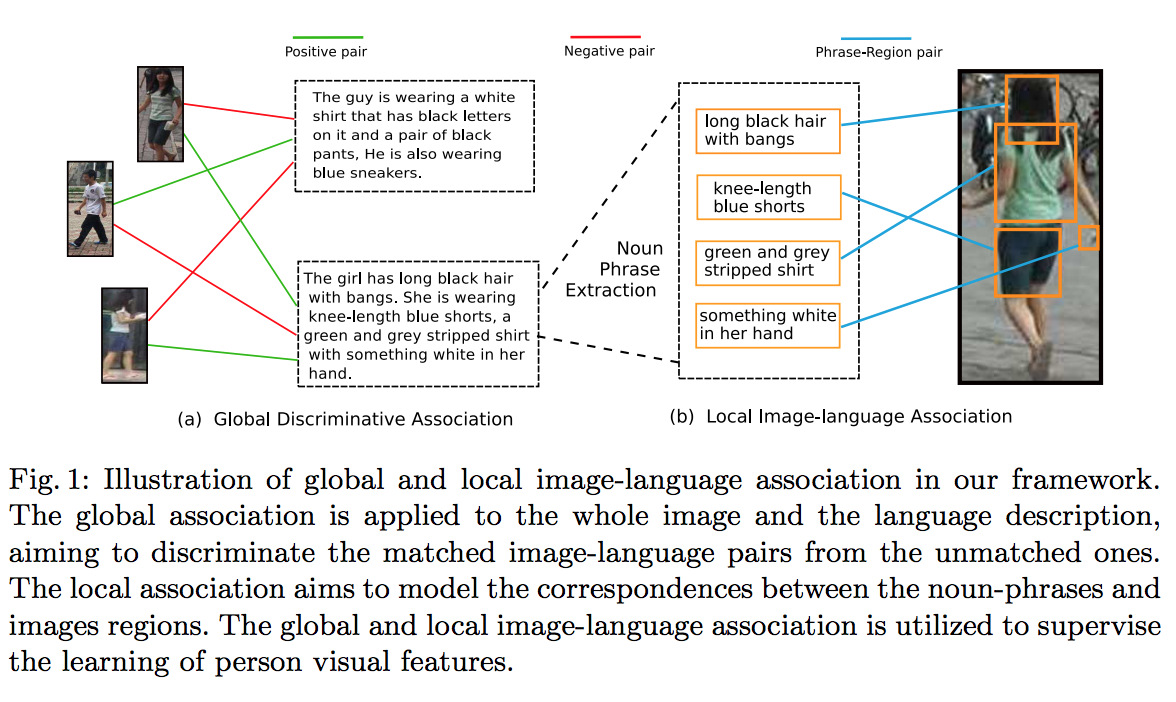
Dapeng Chen et.al., Improving Deep Visual Representation for Person Re-identification by Global and Local Image-language Association, ECCV’18よりFig.1を転載。
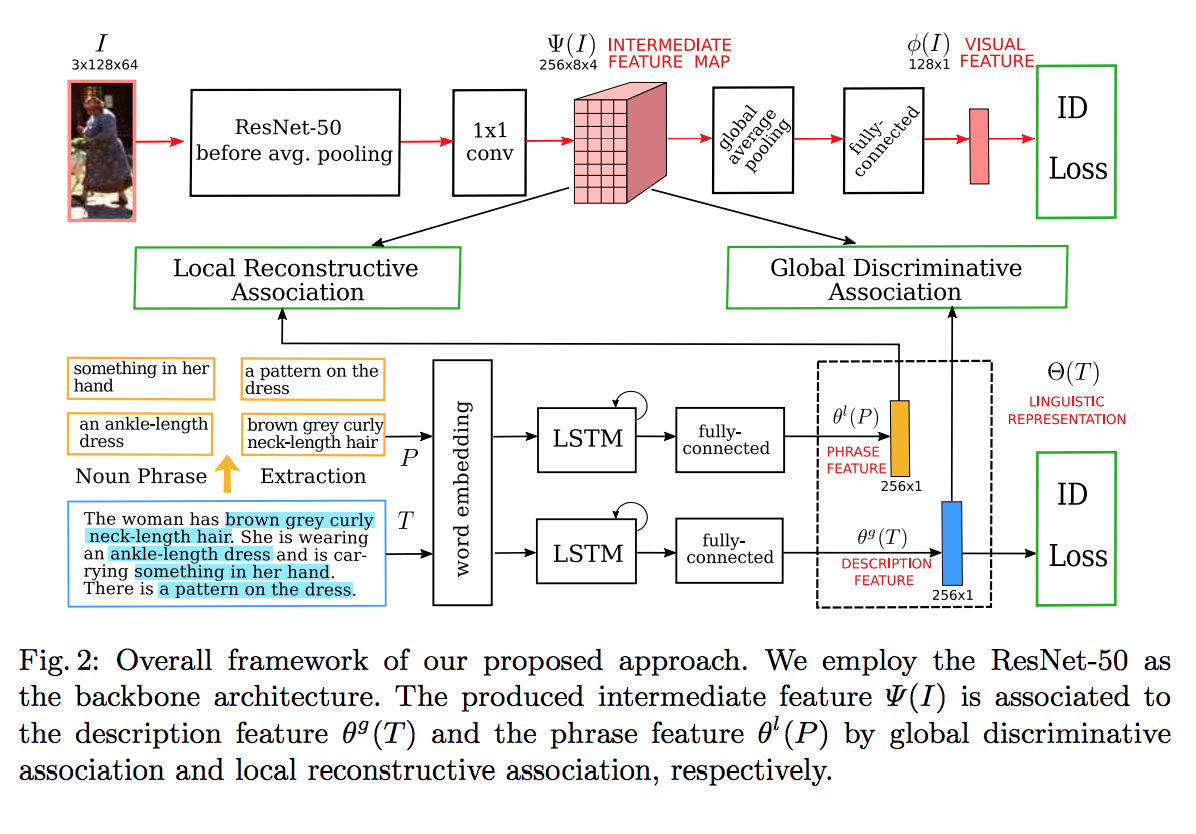
Dapeng Chen et.al., Improving Deep Visual Representation for Person Re-identification by Global and Local Image-language Association, ECCV’18よりFig.2を転載。
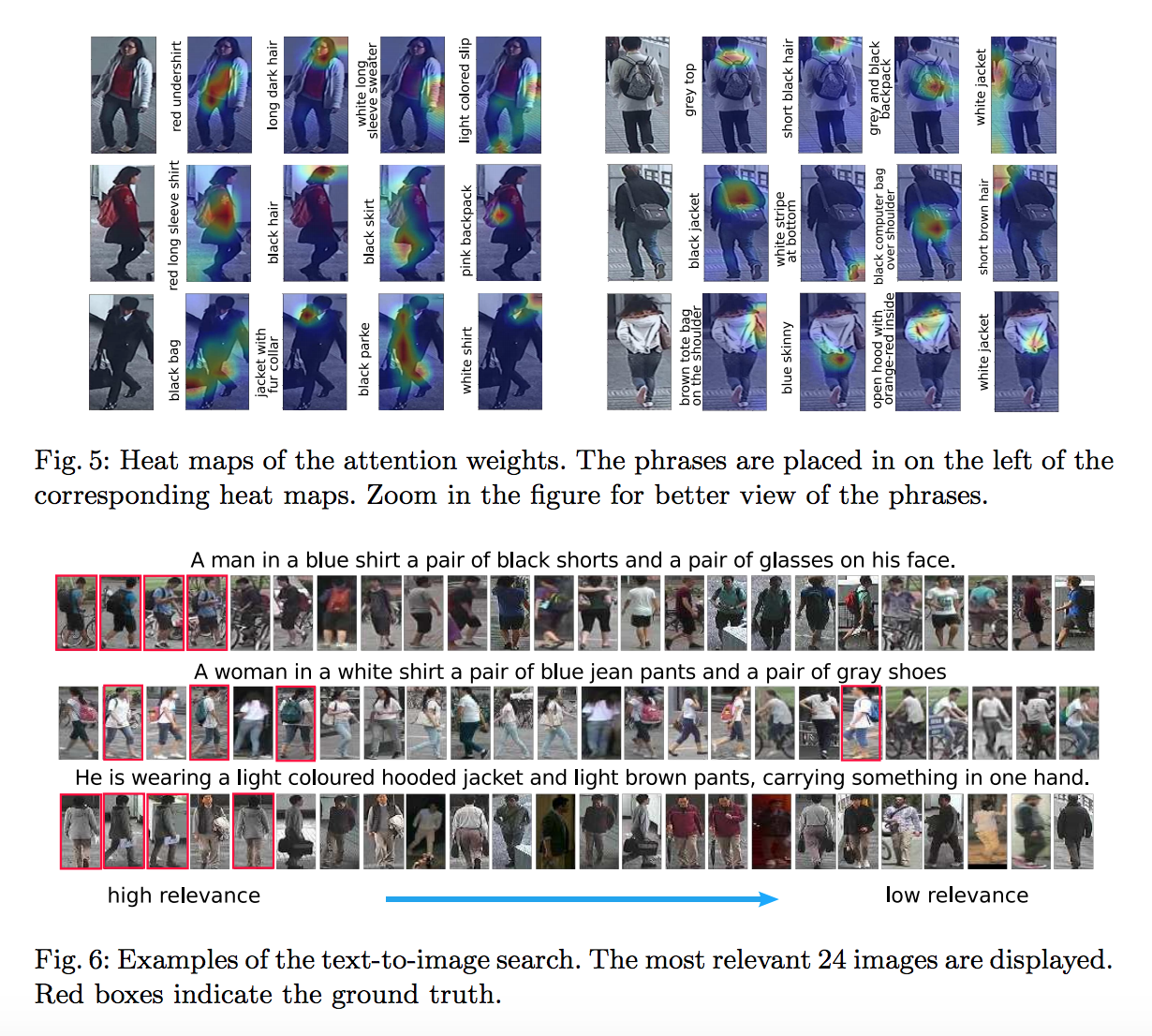
Dapeng Chen et.al., Improving Deep Visual Representation for Person Re-identification by Global and Local Image-language Association, ECCV’18よりFig.6を転載。
上記の論文では、画像に映っている複数の物体(事物)間の(セマンティックな)関係(物理空間内の配置関係など)を推定して、画像に収められている光景が、どのような意味(セマンティクス)を帯びた「シーン(Scene)」であるのかを理解する(Scene understanding task)モデルが提案されています。
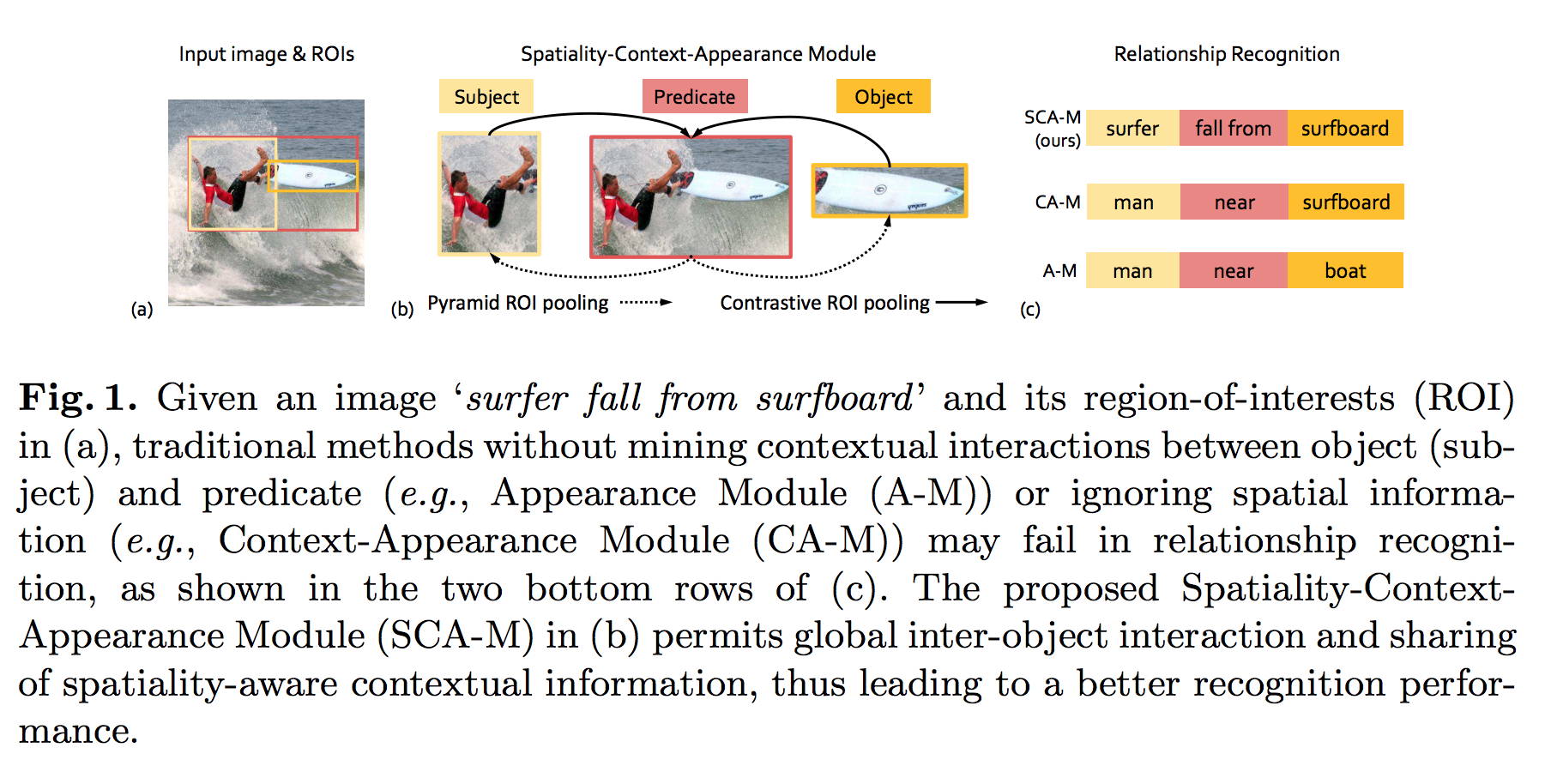
Guojun Yin et.al., Zoom-Net: Mining Deep Feature Interactions for Visual Relationship Recognition, ECCV’18よりFig.1を転載。
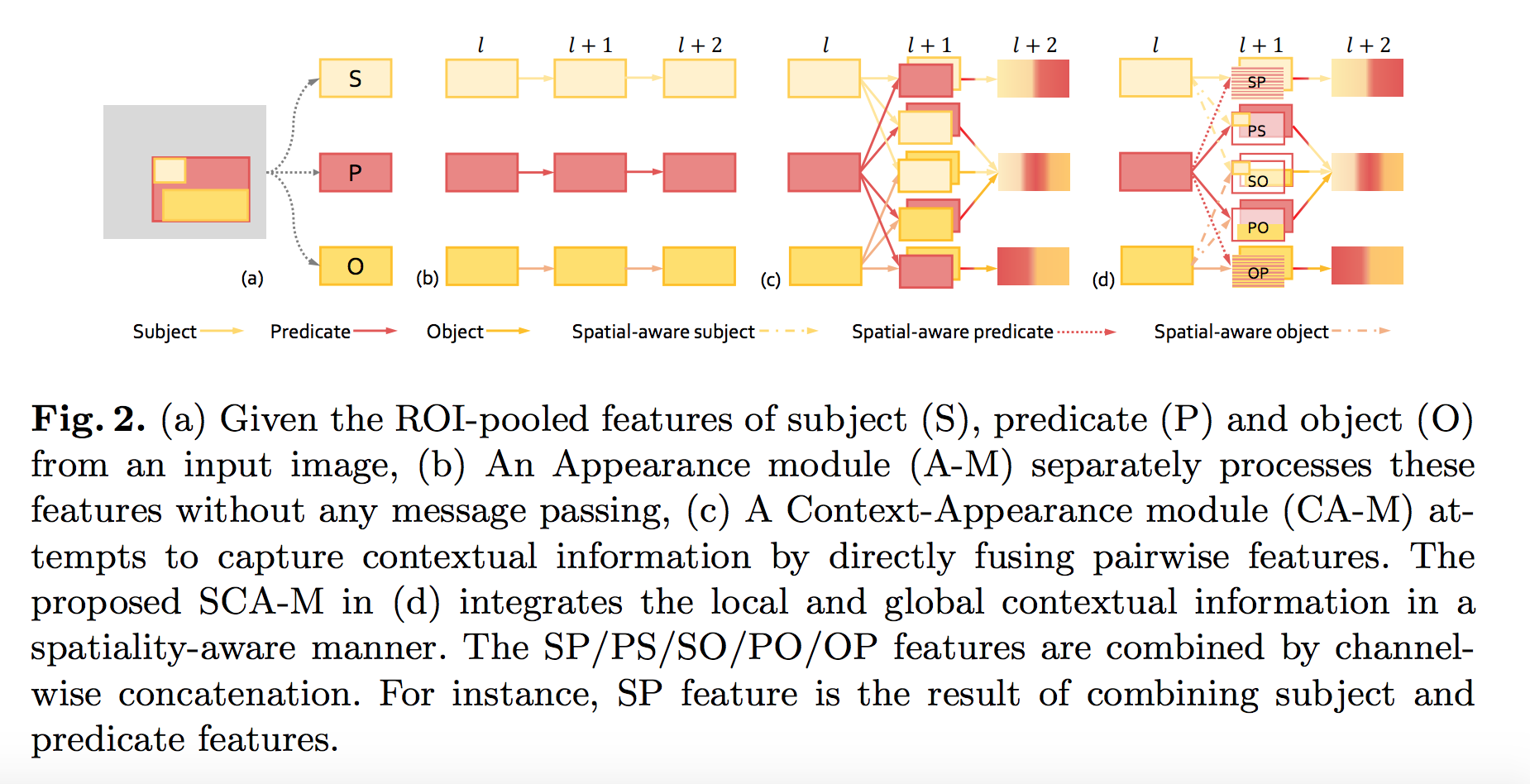
Guojun Yin et.al., Zoom-Net: Mining Deep Feature Interactions for Visual Relationship Recognition, ECCV’18よりFig.2を転載。
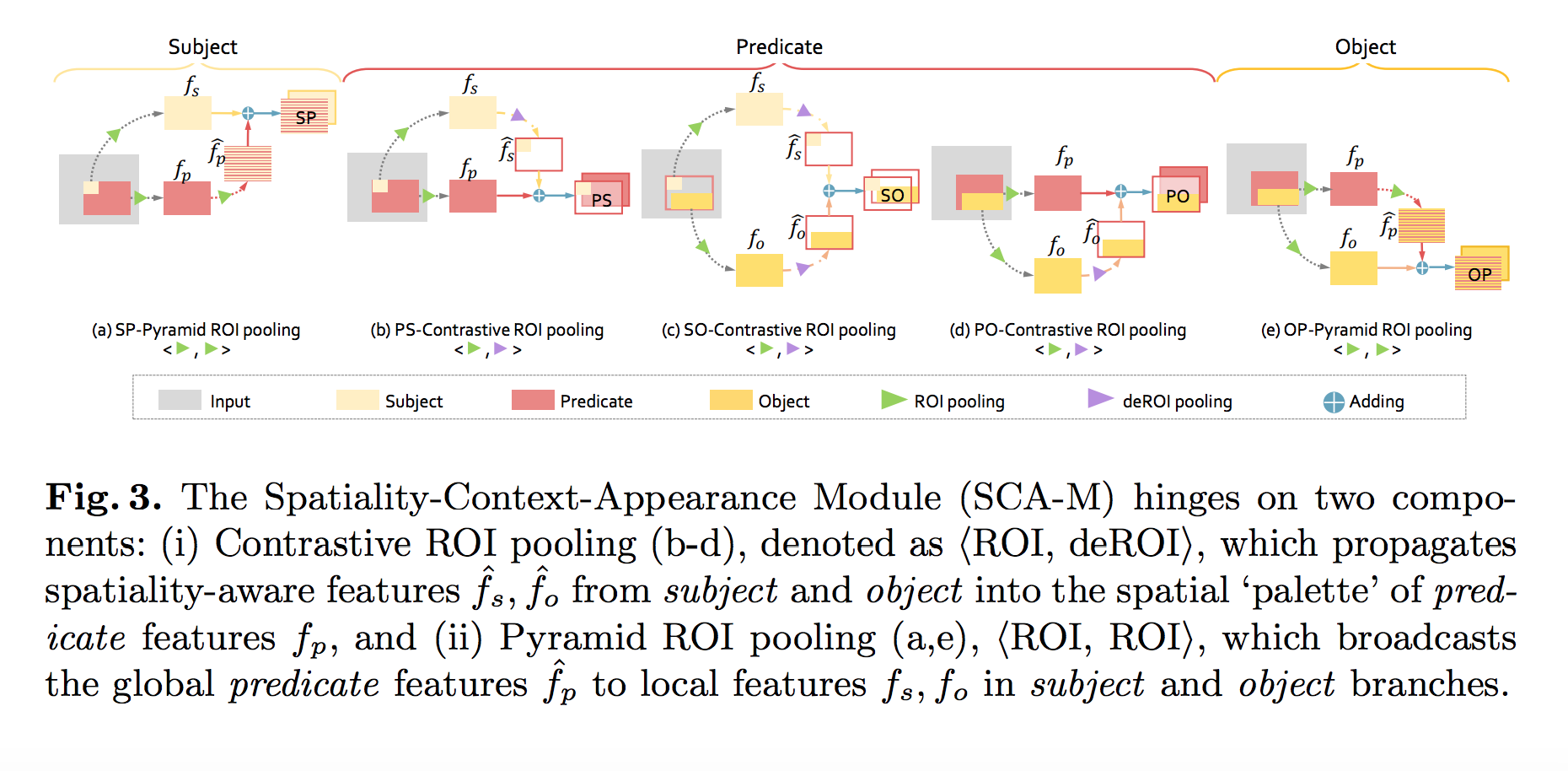
Guojun Yin et.al., Zoom-Net: Mining Deep Feature Interactions for Visual Relationship Recognition, ECCV’18よりFig.3を転載。
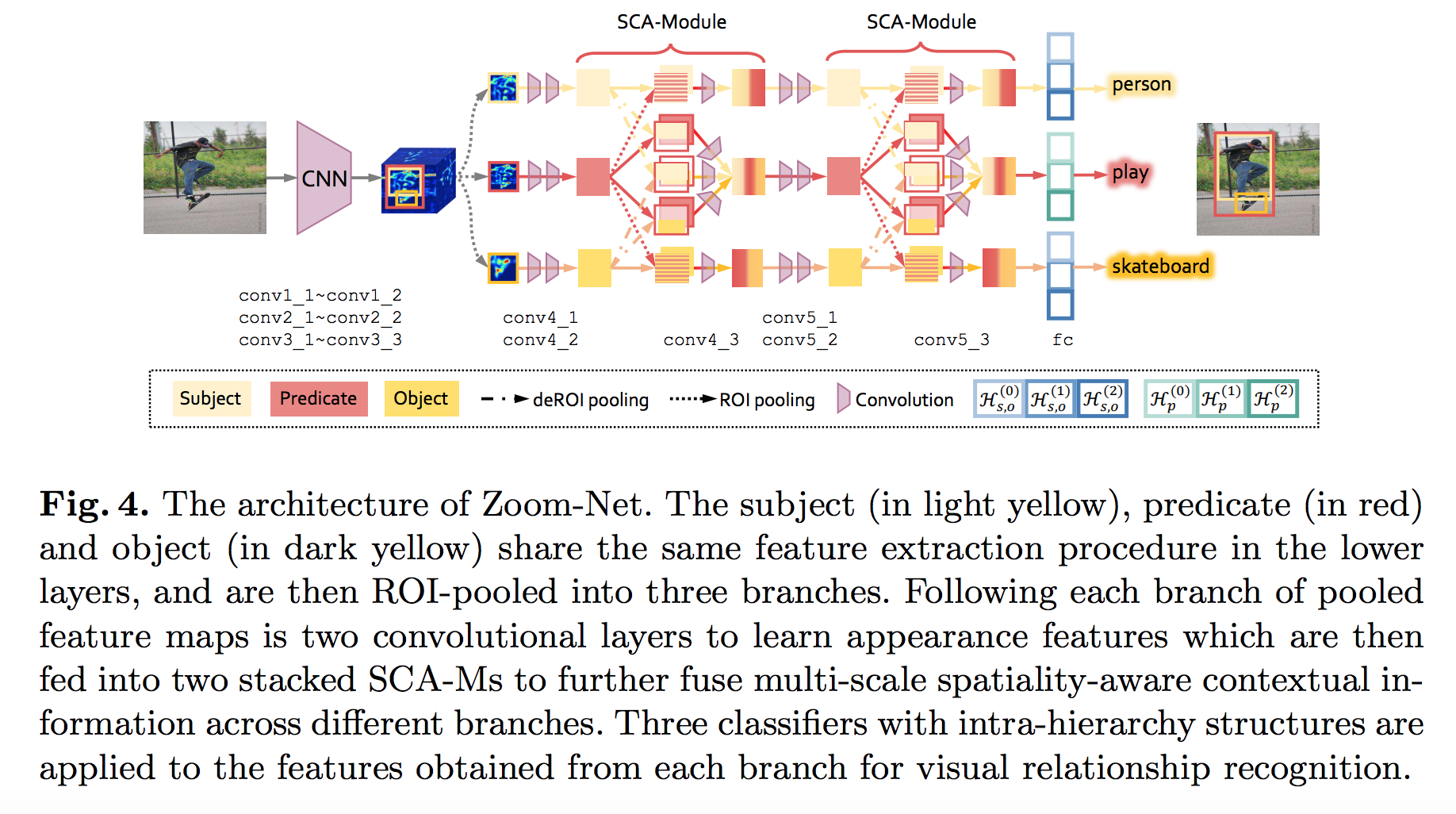
Guojun Yin et.al., Zoom-Net: Mining Deep Feature Interactions for Visual Relationship Recognition, ECCV’18よりFig.4を転載。
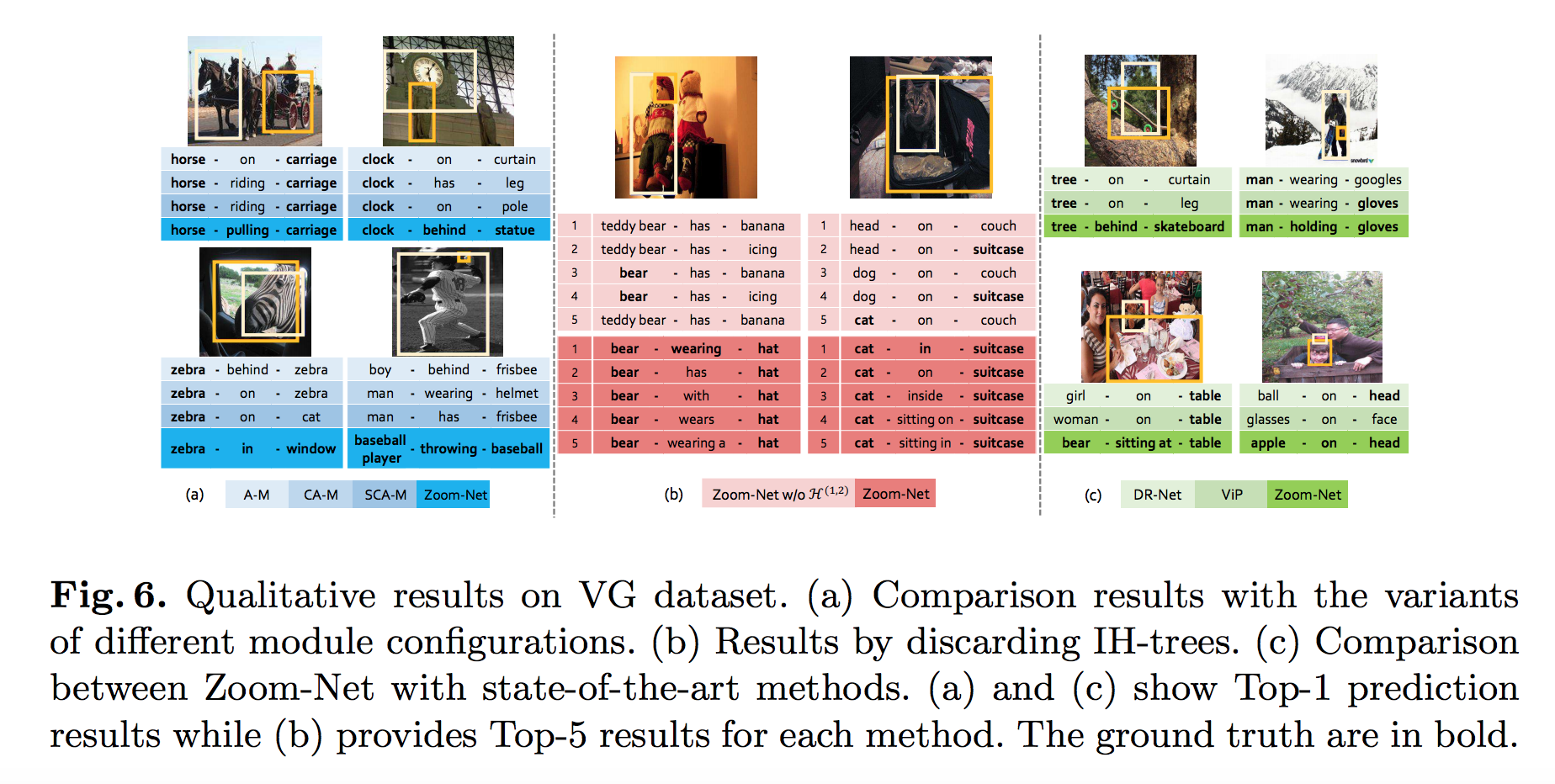
Guojun Yin et.al., Zoom-Net: Mining Deep Feature Interactions for Visual Relationship Recognition, ECCV’18よりFig.6を転載。
【 注目企業2】メグビー・テクノロジー社(Megvii Technology Inc.,北京曠視科技)
日本ではまだ、センスタイムほどは知られていませんが、画像解析エンジン 「Face++」を生み出したメグビー社も、いま産業界や投資銀行から注目を集めている中国の画像解析テクノロジー・ベンチャー企業です。
この会社は、2011年、清華大学の学生であった印 奇氏(現在、CEO)と唐 文斌氏(現在、CTO)が創業し、のちに、杨 沐氏が合流して3人で始めた学生ベンチャー企業として始まったようです。
- Megvii社 印 奇CEO
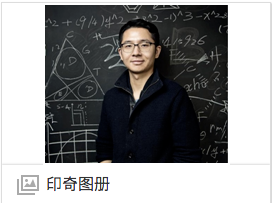
Baiduより転載。 https://baike.baidu.com/item/%E5%8D%B0%E5%A5%87
- Megvii社 唐 文斌CTO
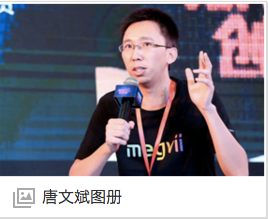
Baiduより転載。 https://baike.baidu.com/item/%E5%94%90%E6%96%87%E6%96%8C/14692119
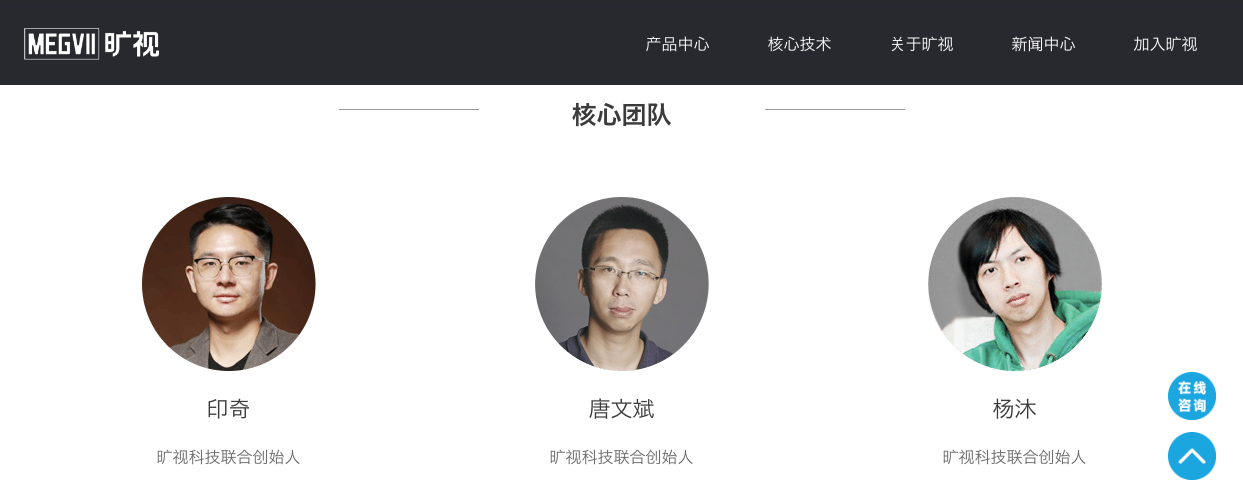
Megvii社公式ウェブページより転載 URL: https://megvii.com/About_Us/
なお、同社内にあるDetection TeamのResearch Leaderは、俞 刚(Gang Yu)氏とのことです。
上記の「略歴(CV)」に記載のあるProfessional Experienceを見ると、2014年12月からMegvii社の本社(北京)に所属(Research Scientist Leader for the Detection team)しているようです。
Megviim社に入社する前の前歴は、以下のようです。
- 2013年5月〜8月まで約半年間:Microsoft Research, Redmond, U.SにてResearch Intern(Advisor: Dr. Zicheng Liu)
- シンガポールのNanyang Technological Universityにて、Research Fellow
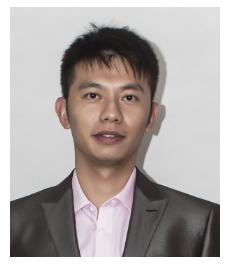
(転載元)http://www.skicyyu.org/
なお、同・経歴書によると、俞 刚氏は、AAAIやCVPRなど、人工知能やComputer Visionの領域で最高峰の国際学会に数多くの論文を査読通過させているようです。
- Chao Peng, Tete Xiao, Zeming Li, Yuning Jiang, Xiangyu Zhang, Kai Jia, Gang Yu, Jian Sun, “MegDet: A Large Mini-Batch Object Detector.” (CVPR), spotlight, 2018. COCO2017 Detection Challenge winner
- Yilun Chen, Zhicheng Wang, Yuxiang Peng, Zhiqiang Zhang, Gang Yu, Jian Sun, “Cascaded Pyramid Network for Multi-Person Pose Estimation.” (CVPR), 2018. COCO2017 Keypoint Challenge winner
- Changqian Yu, Jingbo Wang, Chao Peng, Changxin Gao, Gang Yu, Nong Sang, “Learning a Discriminative Feature Network for Semantic Segmentation.” (CVPR), 2018.
- Zeming Li, Yilun Chen, Gang Yu, Xiangyu Zhang, Jian Sun , “R-FCN++: Towards Accurate Region-based Fully Convolutional Networks for Object Detection.” Association for the Advancement of Artificial Intelligence (AAAI), 2018.
- Chao Peng, Xiangyu Zhang, Gang Yu, Guiming Luo, Jian Sun, “Large Kernel Matters – Improve Semantic Segmentation by Global Convolutional Network.” IEEE Conference on Computer Vision and Pattern Recognition (CVPR), 2017.
- Gang Yu, Junsong Yuan, “Fast Action Proposals for Human Action Detection and Search.” IEEE Conference on Computer Vision and Pattern Recognition (CVPR), 2015.
- Gang Yu, Zicheng Liu, Junsong Yuan, “Discriminative Orderlet Mining For Realtime Recognition of Human-Object Interaction.” Asian Conference on Computer Vision (ACCV), 2014.
- Gang Yu, Junsong Yuan, “Scalable Forest Hashing for Fast Similarity Search.” International Conference on Multimedia and Expo (ICME), 2014.
- Gang Yu, Junsong Yuan, and Zicheng Liu, “Propagative Hough Voting for Human Activity Recognition.” IEEE Conference on European Conference on Computer Vision (ECCV), 2012.
- Yuning Jiang, Gang Yu, Junsong Yuan, “Randomized Spatial Partition for Scene Recognition.” IEEE Conference on European Conference on Computer Vision (ECCV), 2012.
- Gang Yu, Junsong Yuan, and Zicheng Liu, “Predicting Human Activities using Spatio-Temporal Structure of Interest Points.” ACM Multimedia Conference (ACMMM), 2012.
- Gang Yu, Junsong Yuan, and Zicheng Liu, “Unsupervised Random Forest Indexing for Fast Action Search.” IEEE Conference on Computer Vision and Pattern Recognition (CVPR), 2011.
- Gang Yu, Junsong Yuan, and Zicheng Liu, “Real-time Human Action Search using Random Forest based Hough Voting.” ACM Multimedia Conference (ACMMM), 2011.
- Gang Yu, Zhiwei Hu, and Hongtao Lu, “Robust Incremental Subspace Learning for Object Tracking.” International Conference on Neural Information Processing, 2009.
- Gang Yu, and Hongtao Lu, “Illumination Invariant Object Tracking with Incremental Subspace Learning.” IEEE International Conference on Image and Graphics, 2009.
Megvii社を取り上げた記事
上記のGloTech Trends誌の記事は、Megvii社にまつわる以下の情報を報じています。
- 創業者の印 奇(Yin Qi)氏は、2016年度のForbes誌 30歳以下の世界リーダーランキング(テクノロジー部門)で1位に輝いた。
- 高校から中国の理系トップの大学である清華大学に飛び級で入学(学費免除)。米・コロンビア大学で修士号、米・ハーバード大学で博士課程(中退)に進学後、中国に戻り、Megvii社を設立した。
- Megvii社には、数学オリンピックの金メダル獲得経験者を16名、抱えている。
- 2012年8月にリリースした「Face++」のAPI call累積階数は、2000万回を超えている(ユーザ端末は5億台)。「Face++」developperサイトに登録しているエンジニア数は、6万人に到達。
- 法人顧客である「滴滴出行」は、同サービスを利用して車に乗車しようとする乗客が、運転手が、「滴滴出行」システムに登録済みのドライバーかどうかを顔認証で確認するために、「Face++」を使用。
- FinTech領域では、「アントフィナンシャル」・「中信銀行」・「平安銀行」などの金融機関が、利用者の個人認証に「Face++」を使用している。
- 防犯・指名手配人追跡・犯罪捜査領域では、国務院公安部(警察)と契約している。
- 顔認証以外の領域として、物を認証し識別・同定するサービスである「image++」を検討中。
なお、最後の物体識別・同定システムについては、Megvii社所属の研究者(Jian Sun氏)が執筆陣に名を連ねる以下の論文が関わっている可能性があります。
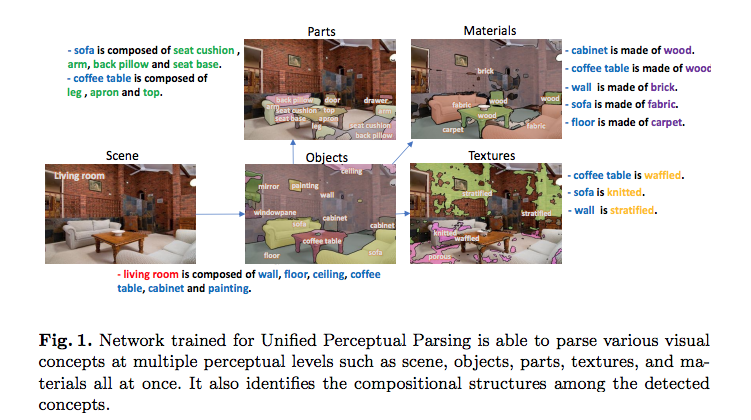
Tete Xiao et.al., Unified Perceptual Parsing for Scene Understanding, ECCV’18より転載。
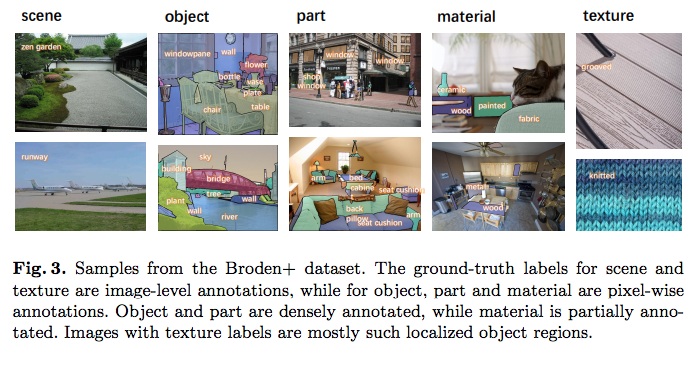
Tete Xiao et.al., Unified Perceptual Parsing for Scene Understanding, ECCV’18より転載。
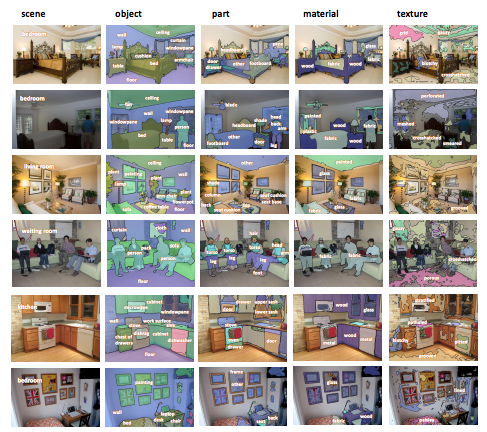
Tete Xiao et.al., Unified Perceptual Parsing for Scene Understanding, ECCV’18より転載。
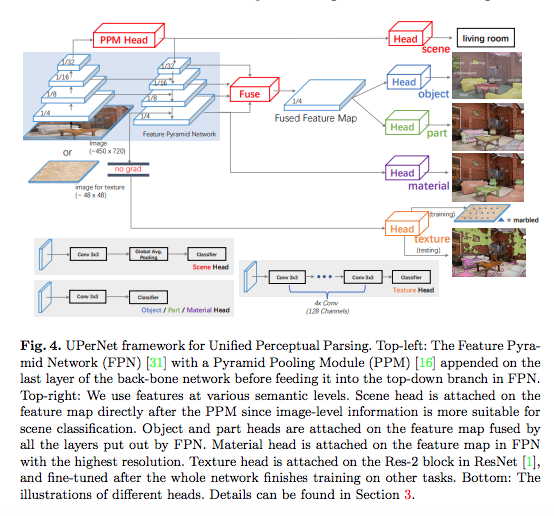
Tete Xiao et.al., Unified Perceptual Parsing for Scene Understanding, ECCV’18より転載。
この論文は、MITの研究者との共同執筆論文です。この論文をMegvii社との絡みで論じている記事としては、以下があります。
なお、Ainowの新海 拓也氏の記事によると、物体の素材や質感を画像中の該当領域ごとに(semantic segmentationの実行を含めて)カテゴリ推定するタスクは、MIT内のある研究チームが注力して研究している分野です。
MITが、米国企業と共同で行っている以下の一群の研究は、MITの研究者を介して、Megvii社の技術陣と知見の交流が図られている可能性も指摘できます。
Jian Sun氏のウェブページとLinkedInは、以下になります。LinkedInによると、同氏のMegvii社におけるポジションは、Chief Scientist / Managing Director of Researchのようです。
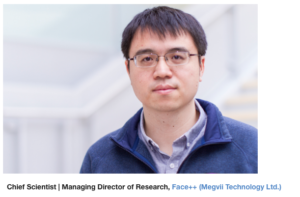
(掲載元URL) http://www.jiansun.org/
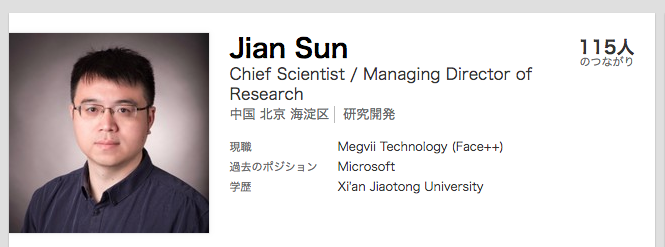
(掲載元URL) https://cn.linkedin.com/in/jian-sun-264252111
Google scholarの集計では、(2019年2月4日現在)h-indexは76で、i10-indexは、168です(2014年以降に公開した論文のみに絞ると、h-index:68, i10-index:159)
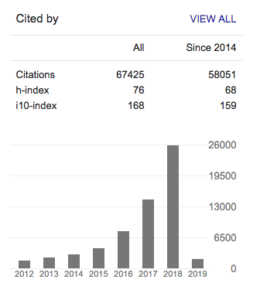
Google Scholar 2019年2月4日現在 https://scholar.google.com/citations?user=7aQ_YLwAAAAJ&hl=en
COURRIER誌も、Megvii社を取り上げています。
中国政府は2016年から、市民監視システム「雪亮工程」(犯罪を未然に防ぎ、雪のように白く輝くクリーンな社会の実現を目指すプロジェクトの意)を推し進めている。顔認証システムや車のナンバープレート照合システムを活用し、犯罪者や犯罪予備軍の個人をピンポイントで取り締まる取り組みだ。
雪亮工程の構築に多大な貢献をしているのが「Face++(フェイスプラスプラス)」であることを疑う人はいない。「Face++」は2011年創業の人工知能(AI)ソフトウェアベンチャー、北京曠視科技(メグヴィー・テクノロジー)が独自開発し、顔認証技術にAIを搭載した世界初のシステムだ。非公式情報だが、中国の公安は2017年末時点で、「Face++」によって5000人以上の逃走犯を逮捕したとされる。上海の女を指名手配者データと一致させたのも「Face++」だった。
「Face++」は何がそんなに凄いのか。専用サイトによると、他社技術に比べ、ジェスチャー認識「Gesture Recognition」と目線の認識「Gaze Estimation」の精度が抜きん出ているという。83のデータポイントから顔をトレースするだけではなく、顔の形状や表情、仕草などを総合的に分析してデータベース化し、性別、年齢、人種などを割り出す。特に目線は上や斜めを向いていても個人を特定できるので、挙動不審な人を重点的に監視することも可能だ。
( 中略 )
ちなみに公安当局は、メグヴィーと並ぶディープラーニング技術のトップ企業・商湯科技開発(センスタイム)が開発した画像認識アルゴリズムも監視カメラネットワークに採用している。センスタイムは米マサチューセッツ工科大学(MIT)や米半導体大手のクアルコム、本田技研工業などとも共同で研究を進め、2018年9月にはソフトバンクから10億米ドル(約1090億円)を調達した。
また、以下の記事もあります。
米マサチューセッツ工科大学(MIT)が刊行する科学技術誌「MITテクノロジー・レビュー」が顔認証決済システムを「10大革新技術」に挙げ、中国のあるスタートアップに注目している。 その企業とは、顔認証ソフトウェアである「フェイスプラスプラス(Face++:以下フェイス++)」を開発した「メグビー(Megvii Technology Inc)」だ。メグビーは中国で顔認証技術を代表する企業である。
以下が、メグビー社の公式ウェブページのランディング・ページ(https://megvii.com/)です。
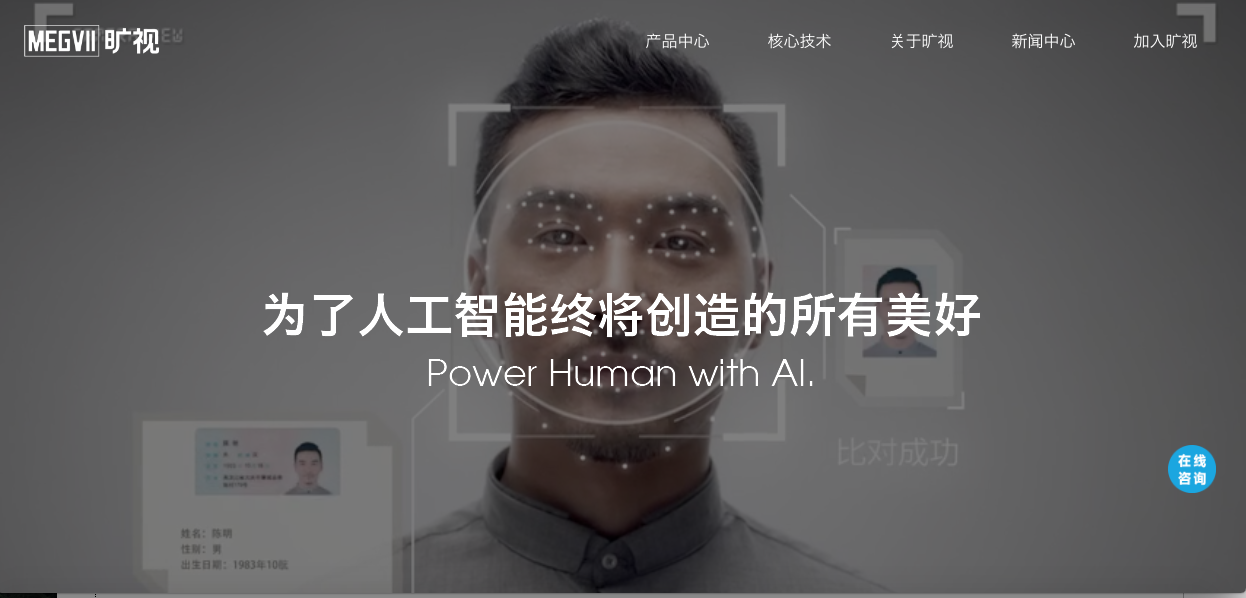
https://megvii.com/
同社は米国国内に研究開発拠点(http://www.megvii.us/)を設置しているようです。
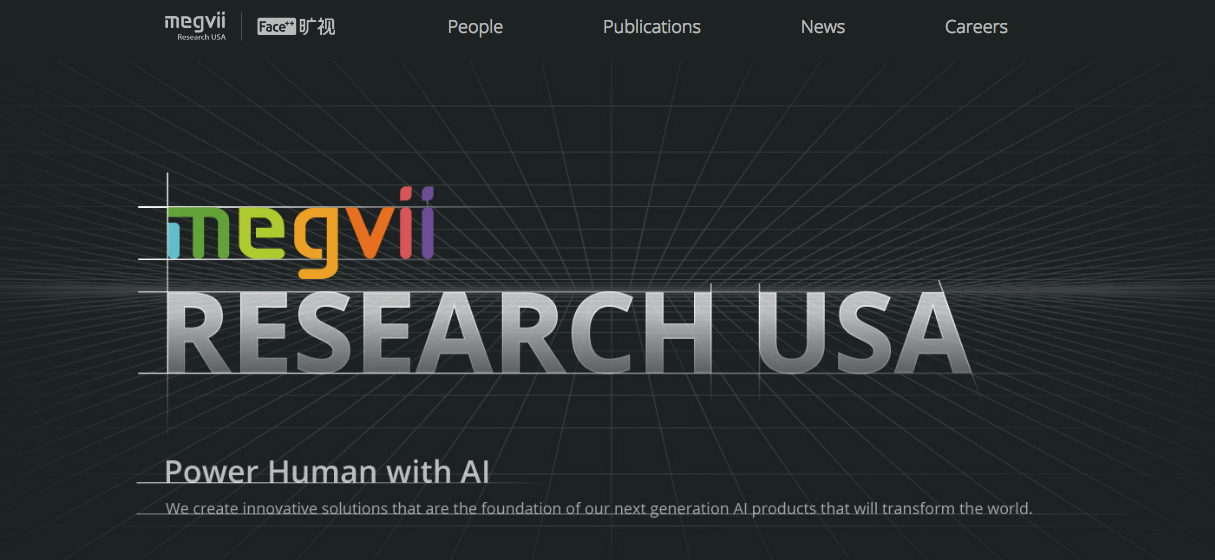
「Face++」の公式ウェブページ(https://www.faceplusplus.com/pricing/)は、以下になります。
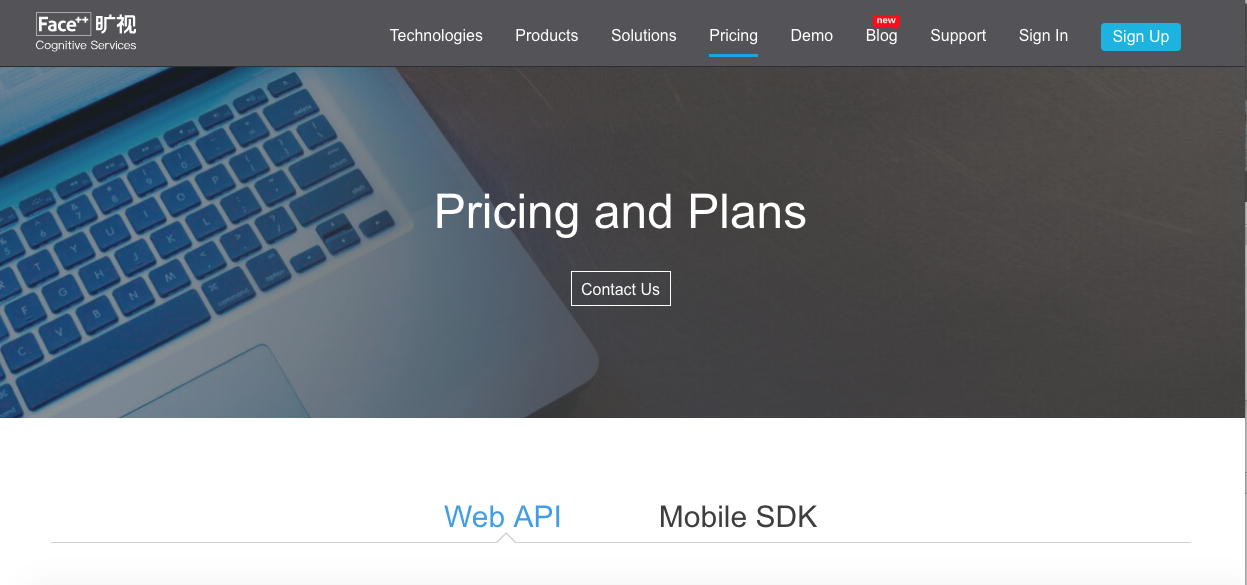
https://www.faceplusplus.com/pricing/
「Face++」の中国語の本家のウェブページ(https://www.faceplusplus.com.cn/)は、以下になります。
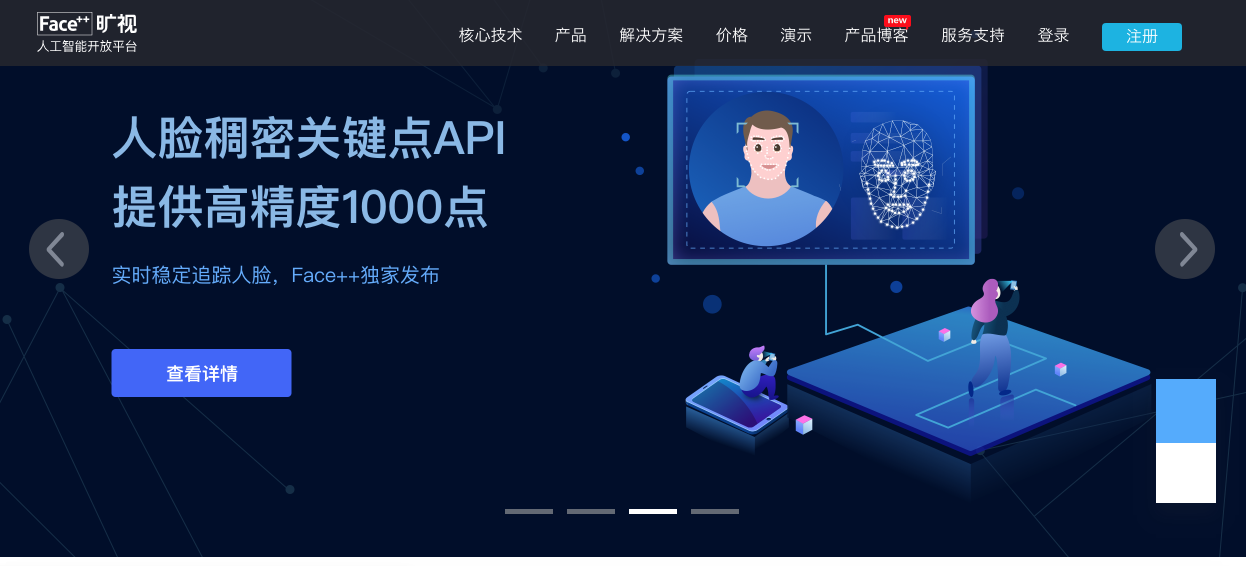
https://www.faceplusplus.com.cn/
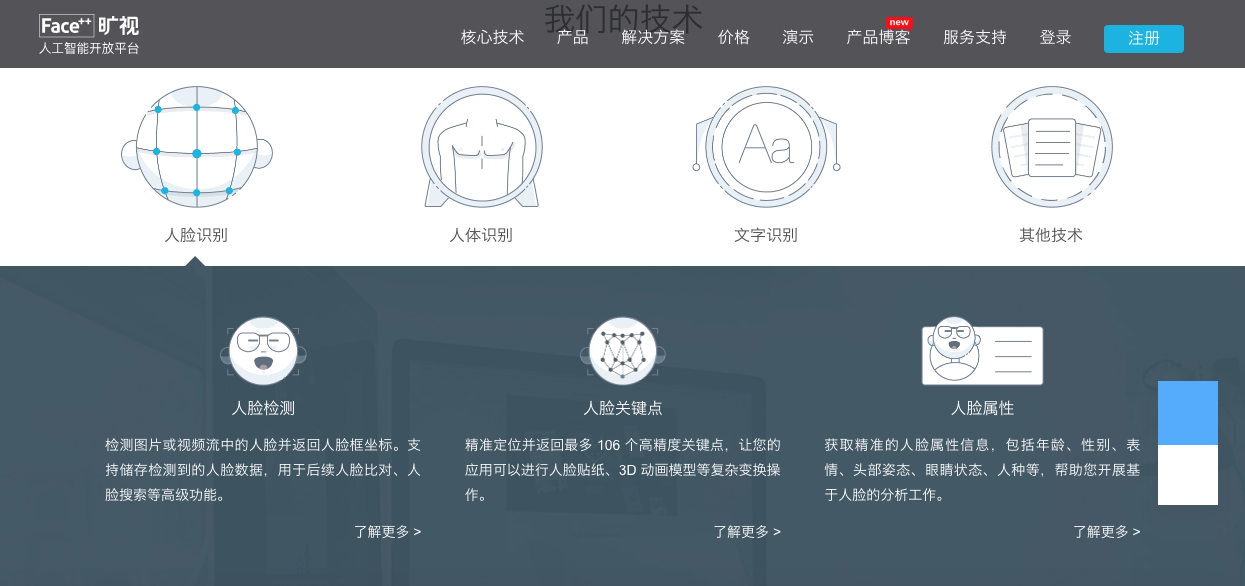
https://www.faceplusplus.com.cn/
- MIT Technology Review by Yiting (Sun 2017.08.16)「日本人が知らない顔認識先進国・中国で躍進する謎のテック企業」
- ROBOTEER 「5000人の逃走犯を検挙した中国 Face++の顔認識AI…その原点はゲームアプリだった」
2011年当時、清華大学の学生だった唐文斌は、同期二人とともに人工知能(AI)の会社を設立する。後に中国を代表する企業へと成長するFace ++(旷视科技)である。
唐文斌は浙江省紹興出身で、幼い頃から「コンピュータの天才」と呼ばれて育った。全国情報オリンピアード大会を総なめにし、高校2年生の時に推薦で清華大学に入学する。中国メディアは、先天的な才能を持って生まれた唐文斌が、清華大学の姚期智教授の専門的な教えを受け、人工知能業界をリードする人材に成長したと分析する。
2010年、唐文斌はマイクロソフトのアジア研究院で実習を行っていた際、横のチームに所属していた印奇と知り合いになる。唐文斌は当時、人間と同様に物事を認識するスマート機器が出現する予測しており、ビジョン認識やセンサーの重要性を感じていたが、印奇もその考えに深く共感したという。二人は構想を具体化・商品化すべく、十分な時間を使う。印奇はフランスに留学。一方の唐文斌は大企業でのノウハウを蓄積する。
その後、AI企業を創業する決定的なきっかけとなったのは、唐文斌と印奇が開発した「Crows Coming(乌鸦来了)」というゲームだった。顔認識技術を活用した同ゲームを運営する過程で、Face ++の共同設立者のひとりである杨沐が合流する。
今回は、Face++が提供するジェスチャー認識「Gesture Recognition」と目線の認識「Gaze Estimation」の精度を実際に試してみました。
「Gesture Recognition」 ── 19種類のジュスチャーに分類する
ジェスチャーを認識するGesture API。19種類のジェスチャーに対応した学習モデルから、画像のジェスチャーが何であるか判定します。
Megvii社から公開されている論文(一部)
この会社からも、多くの論文が公開されています。前述の俞 刚氏の論文を含めて、同社から出ている論文(一部)を掲載します。
- Changqian Yu et.a., BiSeNet: Bilateral Segmentation Network for Real-time Semantic Segmentation, ECCV’18
- Haoyue Shi et.al., Learning Visually-Grounded Semantics from Contrastive Adversarial Samples, International Conference on Computational Linguistics 2018
- Chao Peng et.al., MegDet: A Large Mini-Batch Object Detector, Arxiv 2018
- Jianfeng Wang, Ye Yuan & Gang Yu, Face Attention Network: An Effective Face Detector for the Occluded Faces, Arxiv 2017
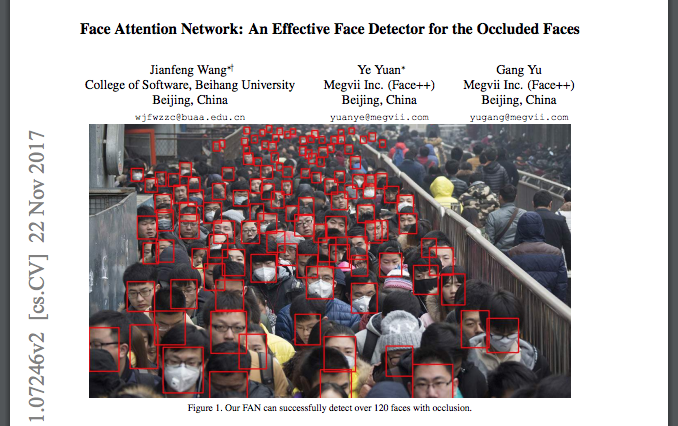
Jianfeng Wang, Ye Yuan & Gang Yu, Face Attention Network: An Effective Face Detector for the Occluded Faces, Arxiv 2017より転載。
- Yilun Chen et.al., Cascaded Pyramid Network for Multi-Person Pose Estimation, Arxiv 2018
- Zeming Li et.al., Light-Head R-CNN: In Defense of Two-Stage Object Detector, Arxiv 2017
- Jianfeng Wang et.al., SFace: An Efficient Network for Face Detection in Large Scale Variations, 2018
- Hexiang Hu et.al., FastMask: Segment Multi-scale Object Candidates in One Shot, Arxiv 2017
- Haoqiang Fan et.al., Learning Deep Face Representation, Arxiv 2014
- Zhaowei Cai et.al., Deep Learning with Low Precision by Half-wave Gaussian Quantization
- [スライド] MSCOCO & PLACES Challenges 2017 Megvii (Face++) Team
上記のほか、このMegvii Inc.に所属する研究者が執筆・公開した論文としては、以下があります。
Abstract. In this paper, we propose a method, called GridFace, to reduce facial geometric variations and improve the recognition performance. Our method rectifies the face by local homography transformations, which are estimated by a face rectification network. To encourage the image generation with canonical views, we apply a regularization based on the natural face distribution. We learn the rectification network and recognition network in an end-to-end manner. Extensive experiments show our method greatly reduces geometric variations, and gains significant improvements in unconstrained face recognition scenarios.
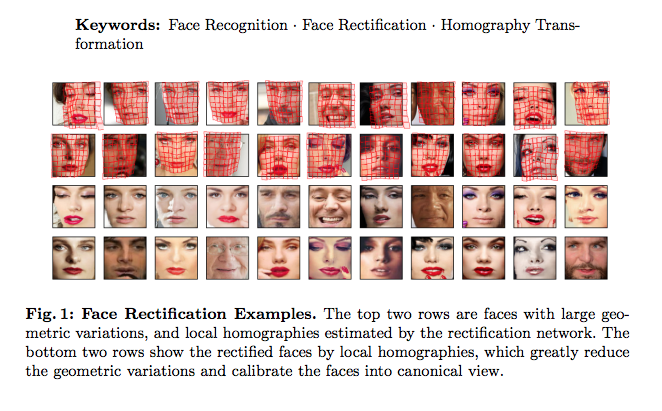
Erjin Zhou, Zhimin Cao, & Jian Sun, GridFace: Face Rectification via Learning Local Homography Transformations, Arxiv 2018より転載。
この論文の筆頭著者であるErjin Zhou氏がこれまでに執筆してきた論文の履歴と被引用数をGoogle Scholar(2019年2月2日現在)でみたもの(https://scholar.google.com/citations?user=k2ziPUsAAAAJ&hl=en)は、以下になります。
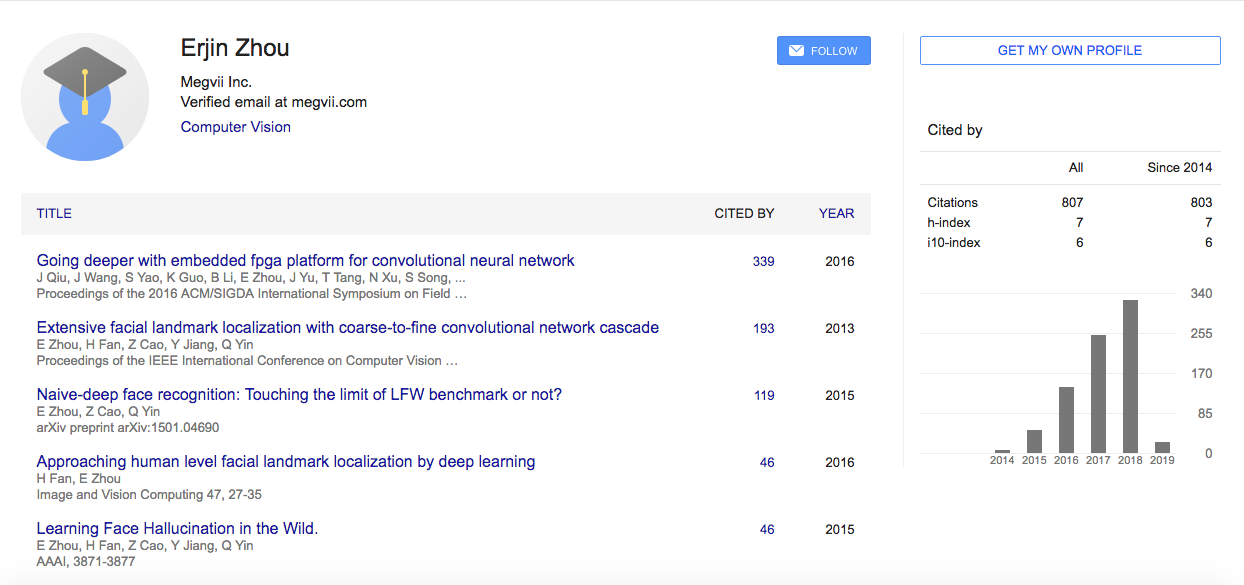
https://scholar.google.com/citations?user=k2ziPUsAAAAJ&hl=en
中国の(動)画像解析技術の研究開発・運用状況を公開論文ベースでみつめていくこの連載記事シリーズでは、Face++をうみだしたMegvii社に所属するErjin Zhou氏の論文も、おいおい取り上げていこうと考えています。
【 注目企業3】ワトリックス社(Watrix,銀河水滴科技)
Watrix社は、黄 永禎(Huang Yongzhen)CEOによって率いられる企業です。
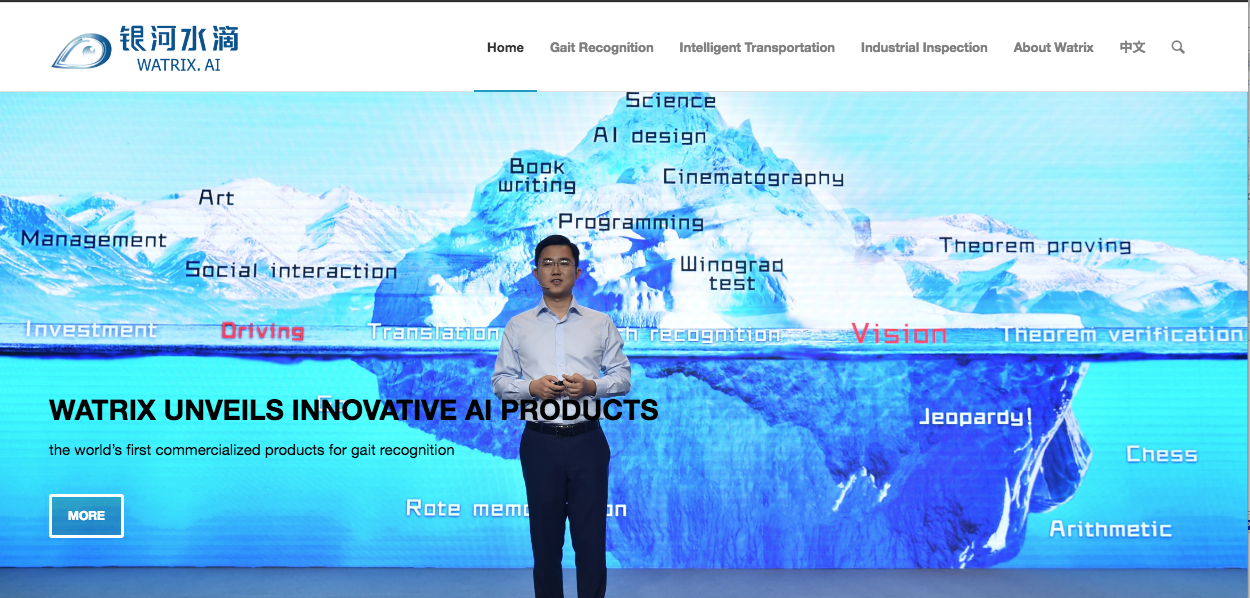
Watrix社公式ウェブページ(英語版) (URL) http://www.watrix.ai/en/
同社の公式ウェブページ(http://www.watrix.ai/en/about-watrix/)によると、経営チームの陣容は以下のとおりです。
- Founder and CEO / Yongzhen Huang博士
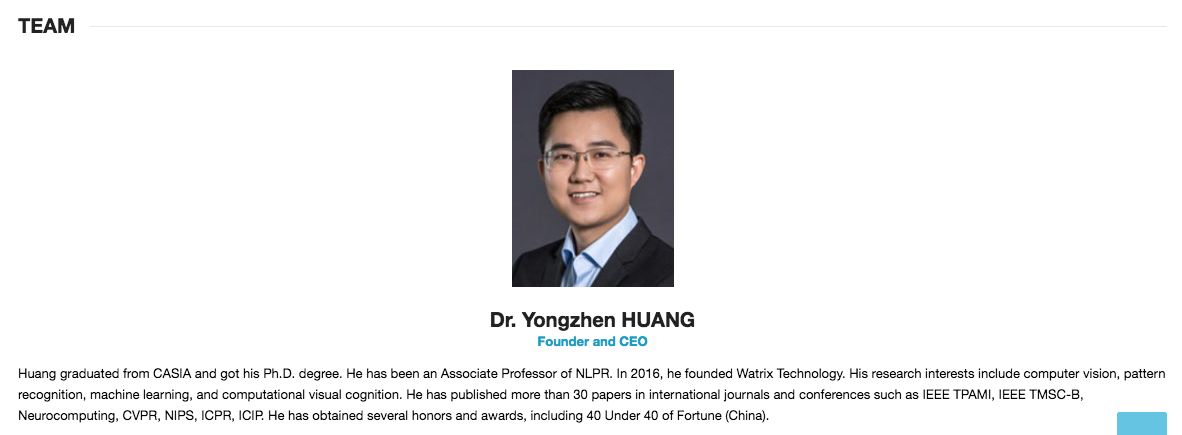
(転載元) http://www.watrix.ai/en/about-watrix/
Huang graduated from CASIA and got his Ph.D. degree.
He has been an Associate Professor of NLPR.
In 2016, he founded Watrix Technology.
His research interests include computer vision, pattern recognition, machine learning, and computational visual cognition.
He has published more than 30 papers in international journals and conferences such as IEEE TPAMI, IEEE TMSC-B, Neurocomputing, CVPR, NIPS, ICPR, ICIP. He has obtained several honors and awards, including 40 Under 40 of Fortune (China).
上記の紹介文から、Watrix社のCEOは研究者として分厚い業績があることが分かります。
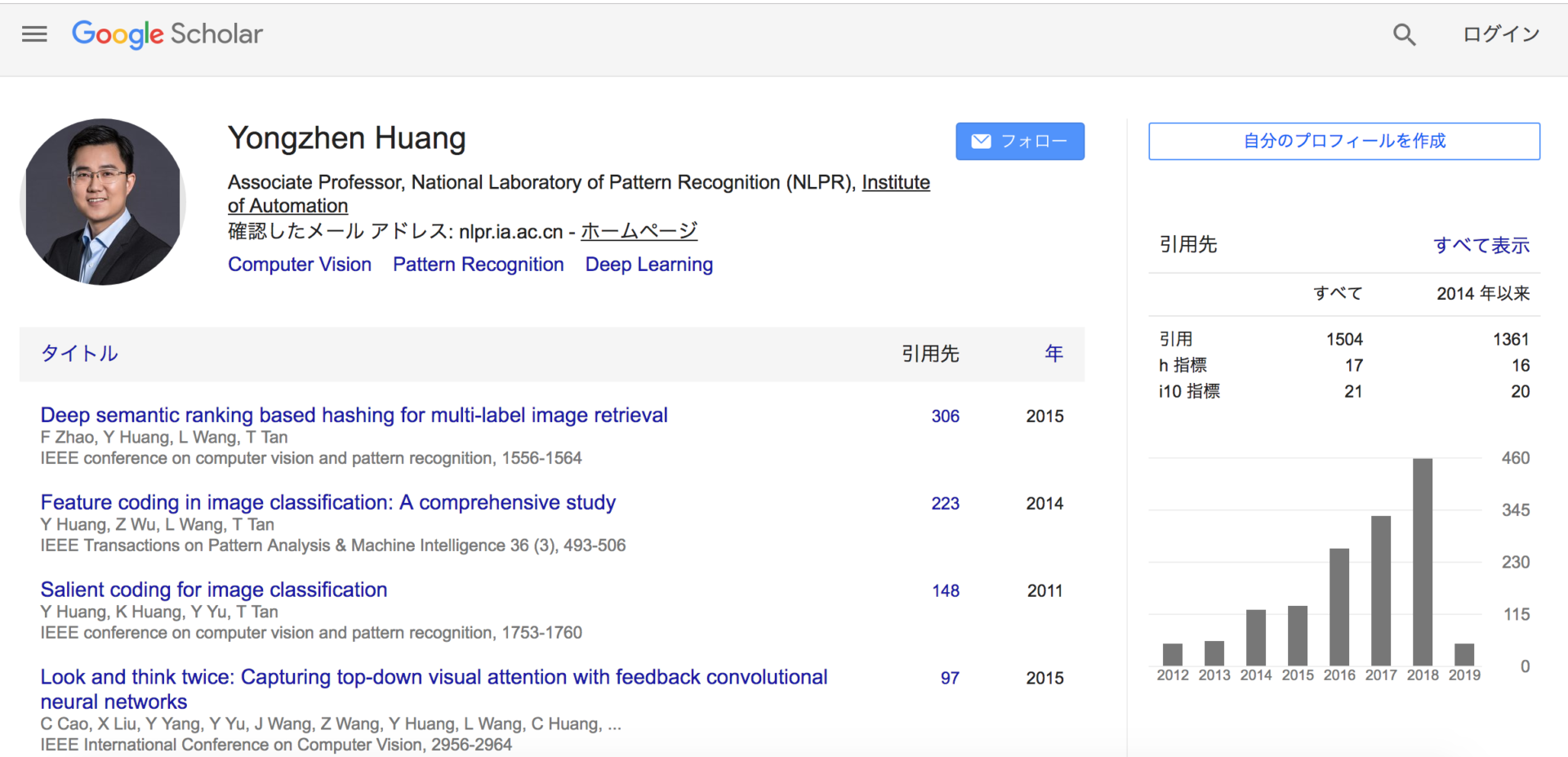
Google Scholar 2019年2月5日現在 URL: https://scholar.google.com/citations?user=OH7-k7oAAAAJ&hl=ja
Google scholarの集計では、(2019年2月5日現在)h-indexは17で、i10-indexは、21です(2014年以降に公開した論文のみに絞ると、h-index:16, i10-index:20)。なお、論文の被引用回数は累計 1,361回と集計されています。
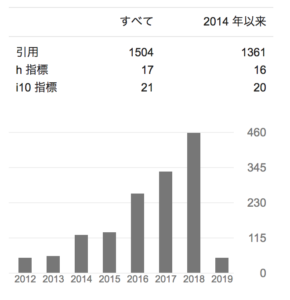
Google Scholar 2019年2月5日現在 URL: https://scholar.google.com/citations?user=OH7-k7oAAAAJ&hl=ja
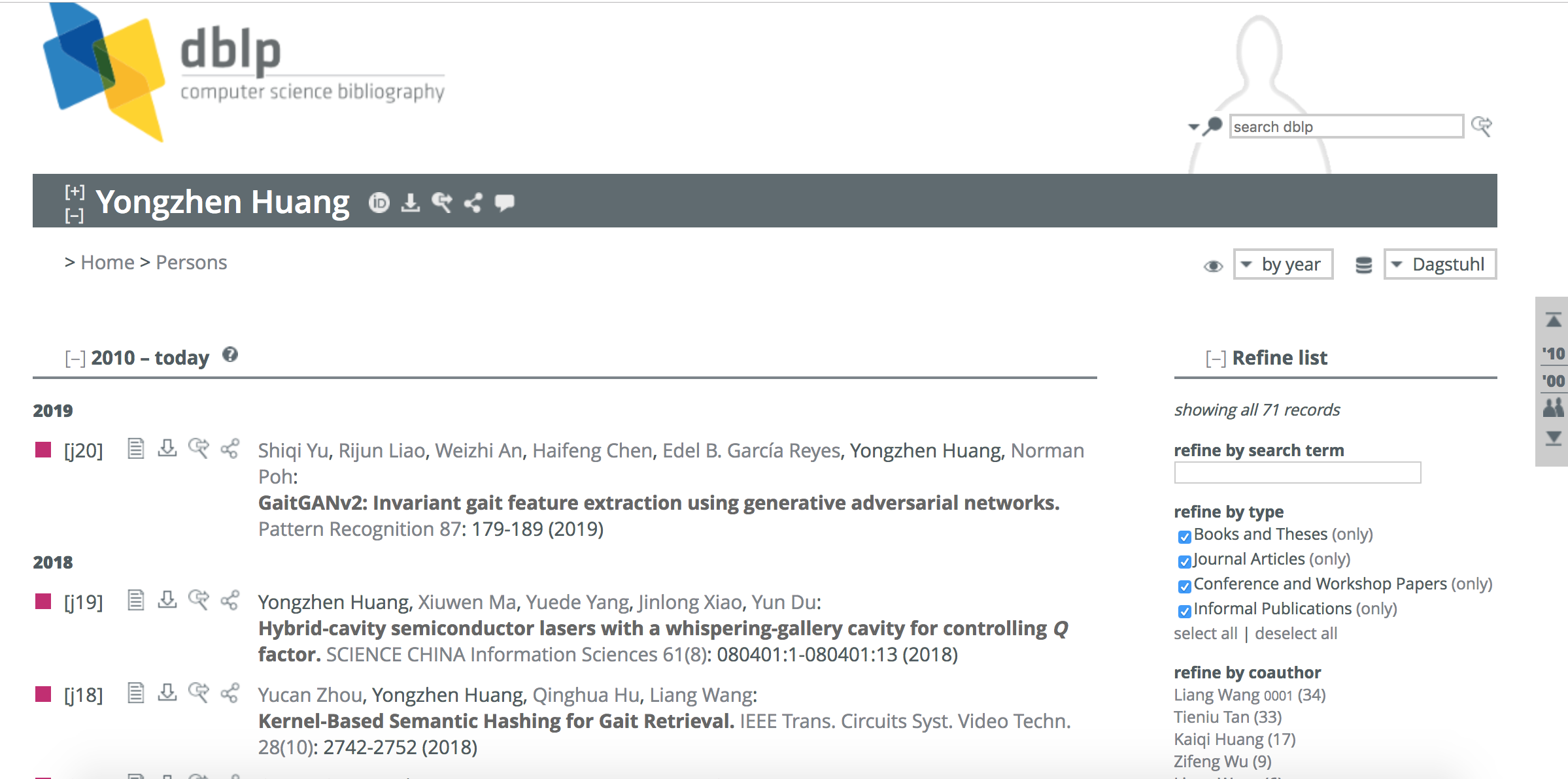
dblp (閲覧日:2019年2月5日) URL: https://dblp.org/pers/hd/h/Huang:Yongzhen
上記のdblpを見ると、直近(2019年2月5日現在)にYongzhen Huang CEOが執筆した論文としては、「足取り」・「歩き方」・「歩様・歩容(ほよう)」を意味する”gait”という単語を含むGaitGANv2モデルの提案論文を公開していることが確認できます。
- Shiqi Yu, Rijun Liao, Weizhi An, Haifeng Chen, Edel B. García Reyes, Yongzhen Huang, Norman Poh: GaitGANv2: Invariant gait feature extraction using generative adversarial networks.Pattern Recognition 87: 179-189 (2019)
- INPST, GaitGANv2: Invariant gait feature extraction using generative adversarial networks
GAN(敵対的生成ネットワーク。Generative Adversarial Networks)モデルによる生成モデルの枠組みで、個人の歩容(Gait)の特徴量、を生成論的に抽出・識別するモデルである可能性を、論文の表題から読み取ることができます。
AbstractThe performance of gait recognition can be adversely affected by many sources of variation such as view angle, clothing, presence of and type of bag, posture, and occlusion, among others. To extract invariant gait features, we proposed a method called GaitGANv2 which is based on generative adversarial networks (GAN).In the proposed method, a GAN model is taken as a regressor to generate a canonical side view of a walking gait in normal clothing without carrying any bag.A unique advantage of this approach is that, unlike other methods, GaitGANv2 does not need to determine the view angle before generating invariant gait images.Indeed, only one model is needed to account for all possible sources of variation such as with or without carrying accessories and varying degrees of view angle.The most important computational challenge, however, is to address how to retain useful identity information when generating the invariant gait images.To this end, our approach differs from the traditional GAN in that GaitGANv2 contains two discriminators instead of one.They are respectively called fake/real discriminator and identification discriminator. While the first discriminator ensures that the generated gait images are realistic, the second one maintains the human identity information.The proposed GaitGANv2 represents an improvement over GaitGANv1 in that the former adopts a multi-loss strategy to optimize the network to increase the inter-class distance and to reduce the intra-class distance, at the same time.Experimental results show that GaitGANv2 can achieve state-of-the-art performance.
以下、この論文のAbstractの冒頭部分では、gait recognition(歩容による個人の識別)の判別精度(performance)は、対象画像に捉えられた被写体の(カメラに対する)角度や着用している衣服の種類、姿勢や、物陰に隠れて見えない領域(「オクルージョン」)などのさまざまな要因によって左右されるため、そのような要因によって左右されない「不変の」・「安定して得られる」(invarient)歩容の特徴を抽出する必要がある。このために、GANモデルの枠組みに基づくGaitGANモデルを提案する、と述べられています。
なお、GaitGANv2の「v2」は、version.2を意味していると思われますが、「v1」に該当するGaitGANモデルは、以下の論文によって提案されているようです。
要約部分の文章は、先に見たGaitGANv2論文の要約文とほぼ変わりません。
Abstract
The performance of gait recognition can be adversely affected by many sources of variation such as view angle, clothing, presence of and type of bag, posture, and occlusion, among others.
In order to extract invariant gait features, we proposed a method named as GaitGAN which is based on generative adversarial networks (GAN).
In the proposed method, a GAN model is taken as a regressor to generate invariant gait images that is side view images with normal clothing and without carrying bags.
A unique advantage of this approach is that the view angle and other variations are not needed before generating invariant gait images.
The most important computational challenge, however, is to address how to retain useful identity information when generating the invariant gait images.
To this end, our approach differs from the traditional GAN which has only one discriminator in that GaitGAN contains two discriminators.
One is a fake/real discriminator which can make the generated gait images to be realistic. Another one is an identification discriminator which ensures that the the generated gait images contain human identification information.
Experimental results show that GaitGAN can achieve state-of-the-art performance. To the best of our knowledge this is the first gait recognition method based on GAN with encouraging results. Nevertheless, we have identified several research directions to further improve GaitGAN.
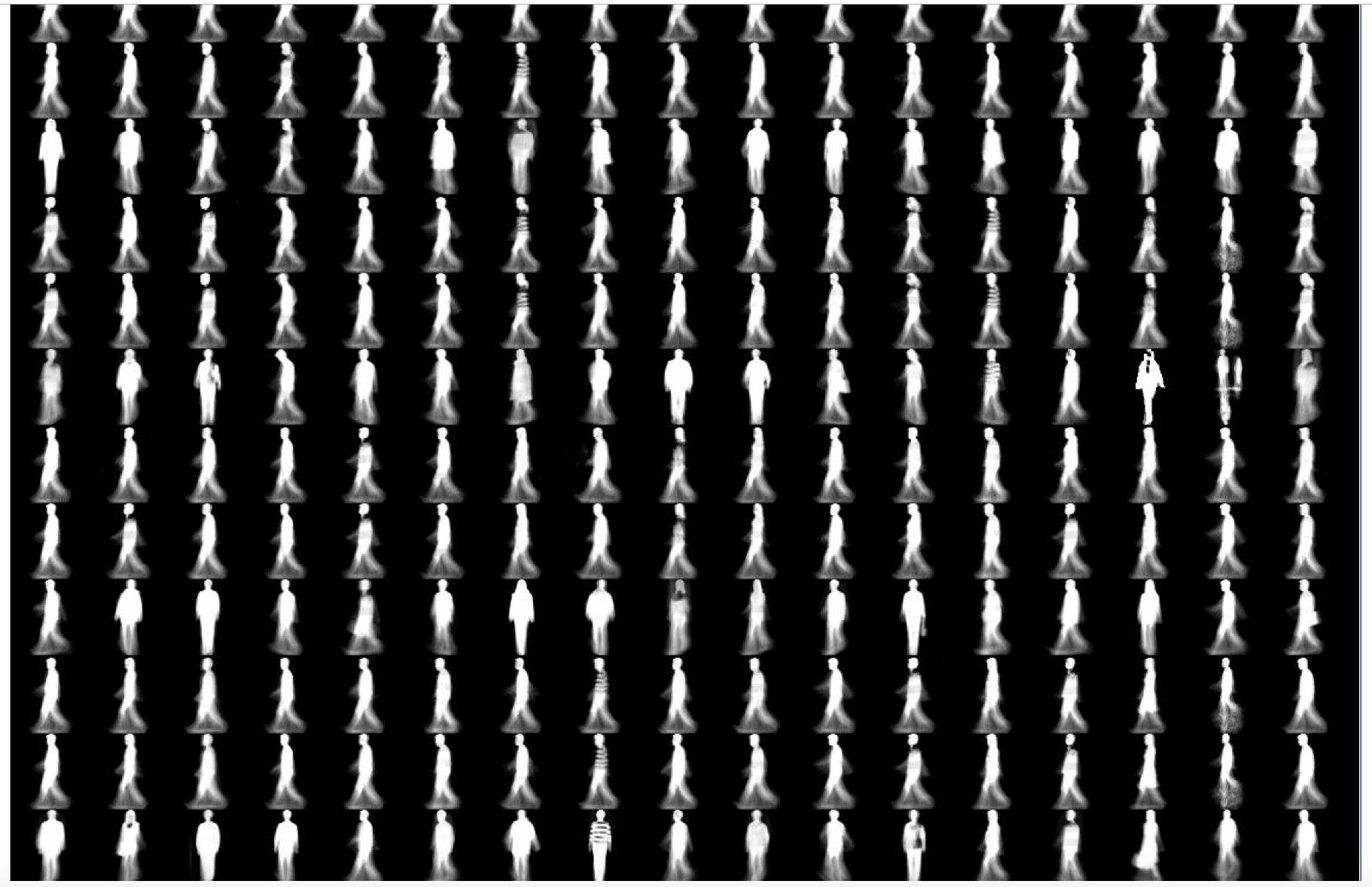
[GitHub] xuehy/pytorch-GaitGAN, GaitGAN: Invariant Gait Feature Extraction Using Generative Adversarial Networksより転載。
- 共同創業者/ Tieniu TAN博士
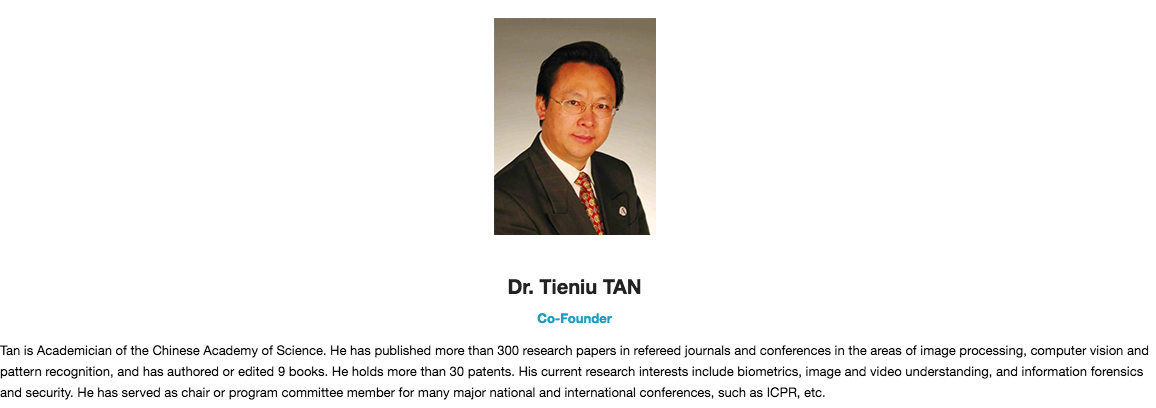
(転載元) http://www.watrix.ai/en/about-watrix/
Tan is Academician of the Chinese Academy of Science.
He has published more than 300 research papers in refereed journals and conferences in the areas of image processing, computer vision and pattern recognition, and has authored or edited 9 books.
He holds more than 30 patents. His current research interests include biometrics, image and video understanding, and information forensics and security.
He has served as chair or program committee member for many major national and international conferences, such as ICPR, etc.
共同創業者であるTieniu TAN博士も、300本もの学術論文をジャーナルや学会誌(Conference)に査読通過させているなど、研究業績が豊かなようです。
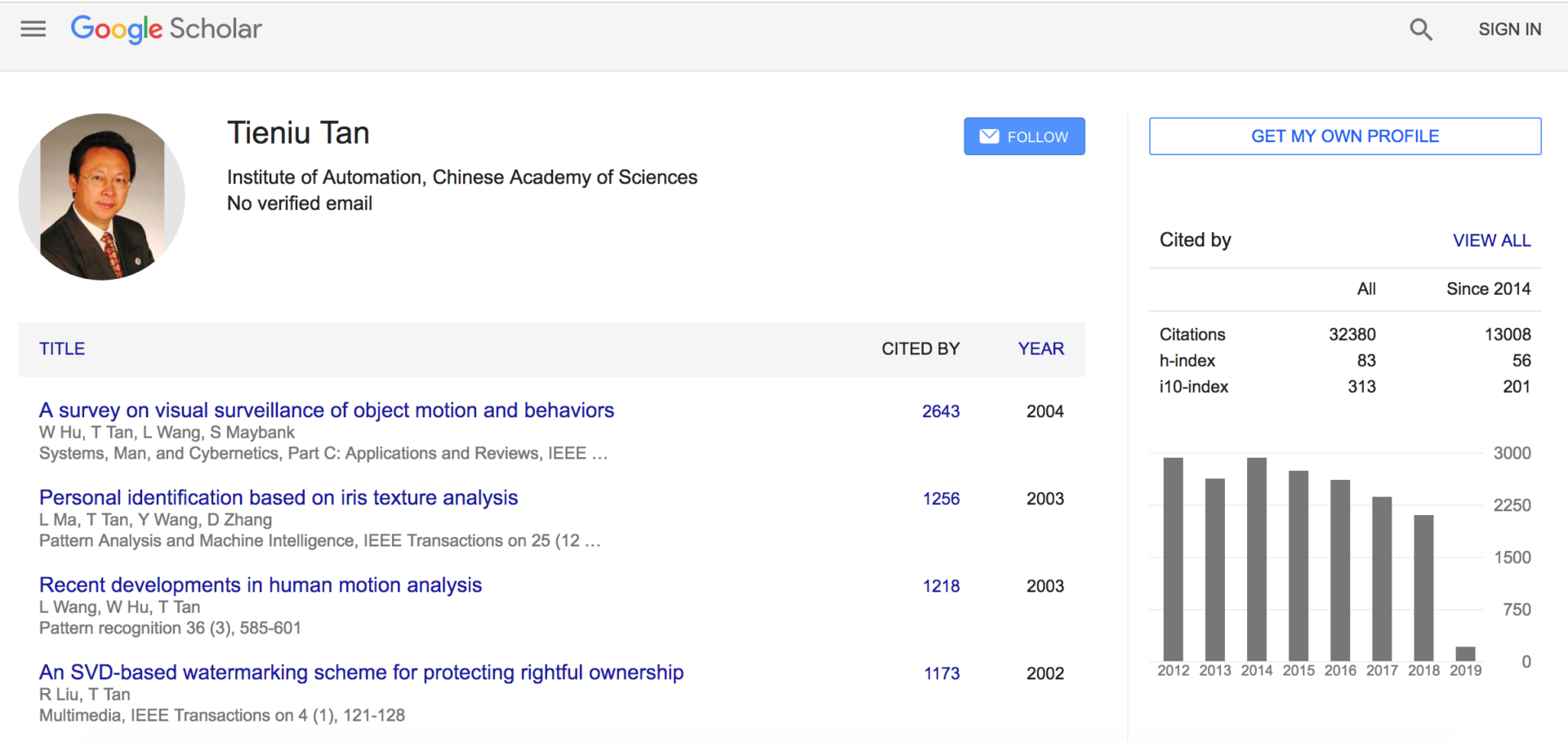
Google Scholar (2019年2月5日現在) URL: https://scholar.google.com/citations?user=W-FGd_UAAAAJ&hl=en
Google scholarの集計では、(2019年2月5日現在)h-indexは83で、i10-indexは、313です(2014年以降に公開した論文のみに絞ると、h-index:56, i10-index:201)。なお、論文の被引用回数は累計 13,008回と集計されています。
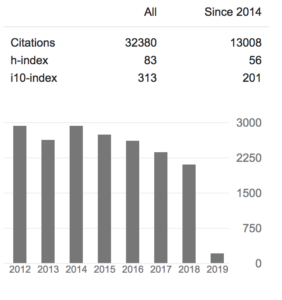
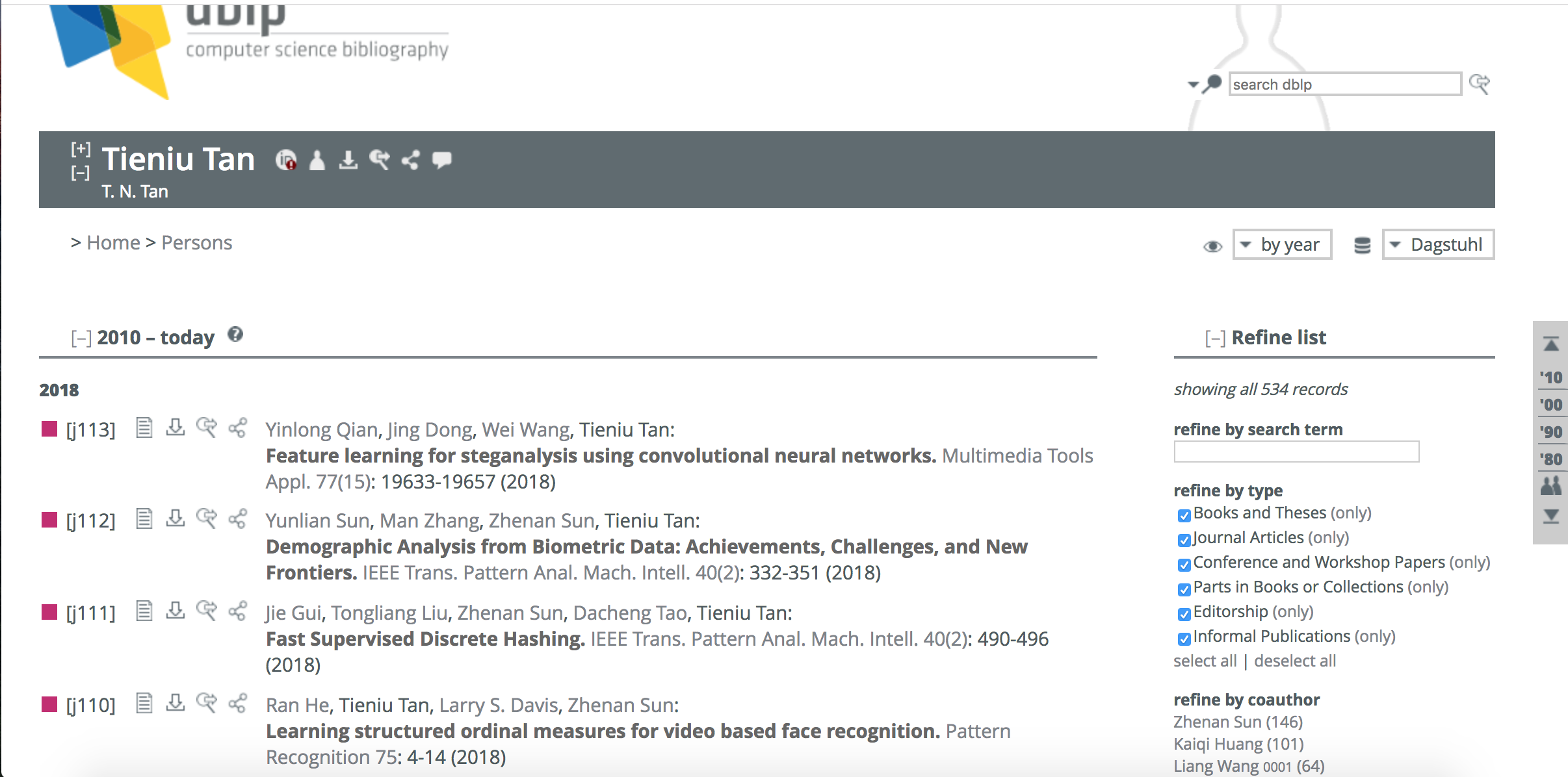
dblp (閲覧日:2019年2月5日) URL: https://dblp.org/pers/hd/t/Tan:Tieniu
同氏も、顔認識や画像の鮮明化(high-resolution)技術にまつわる研究に多く取り組んできた様子が読み取れます。
-
Bo Peng, Wei Wang, Jing Dong, Tieniu Tan:
Image Forensics Based on Planar Contact Constraints of 3D Objects. IEEE Trans. Information Forensics and Security 13(2): 377-392 (2018) -
DeMeshNet: Blind Face Inpainting for Deep MeshFace Verification. IEEE Trans. Information Forensics and Security 13(3): 637-647 (2018)
- Yunlong Wang, Fei Liu, Kunbo Zhang, Guangqi Hou, Zhenan Sun, Tieniu Tan:
LFNet: A Novel Bidirectional Recurrent Convolutional Neural Network for Light-Field Image Super-Resolution. IEEE Trans. Image Processing 27(9): 4274-4286 (2018)
- 共同創業者(兼)アドバイザー/ Liang WANG博士
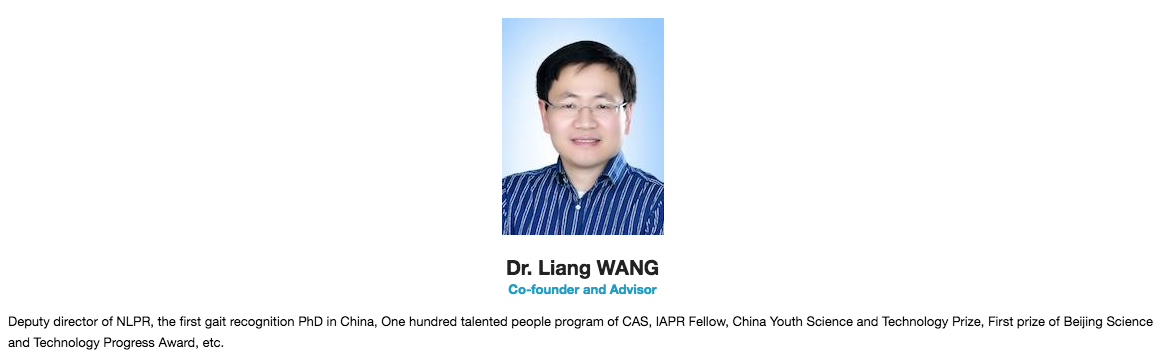
(転載元) http://www.watrix.ai/en/about-watrix/
Deputy director of NLPR, the first gait recognition PhD in China, One hundred talented people program of CAS, IAPR Fellow, China Youth Science and Technology Prize, First prize of Beijing Science and Technology Progress Award, etc.
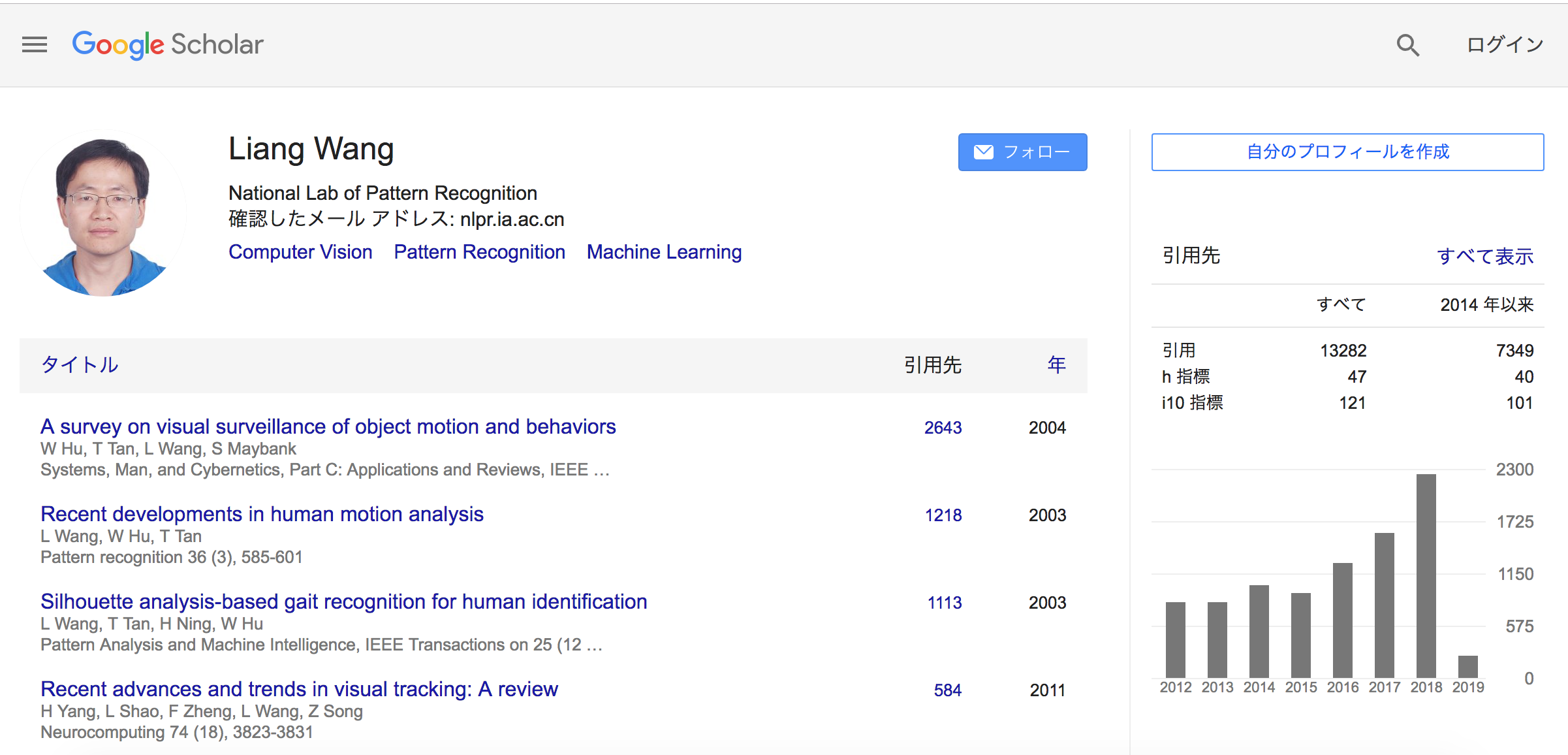
Google Scholar (2019年2月5日調べ) URL: https://scholar.google.com/citations?user=8kzzUboAAAAJ&hl=ja
Google scholarの集計では、(2019年2月5日現在)h-indexは47で、i10-indexは、40です(2014年以降に公開した論文のみに絞ると、h-index:40, i10-index:101)。なお、論文の被引用回数は累計 7,349回と集計されています。
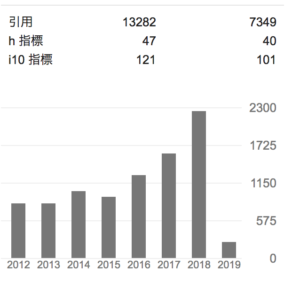
Watrix社が取得した特許(US Patent)
同社の特許としては、以下が見当たりました。
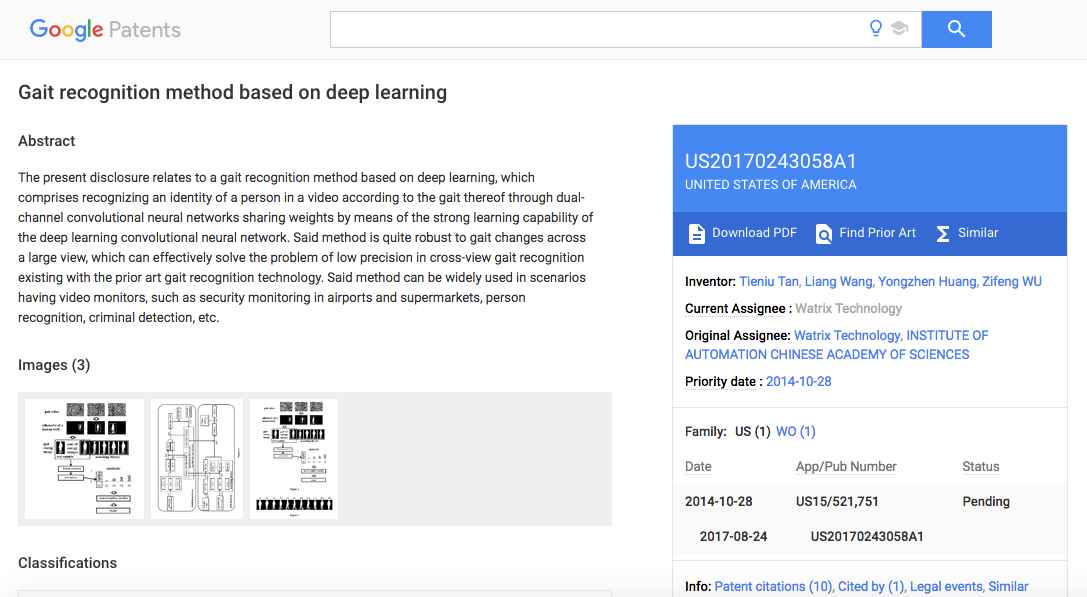
(URL) https://patents.google.com/patent/US20170243058A1/en
Gait recognition method based on deep learning
Abstract The present disclosure relates to a gait recognition method based on deep learning, which comprises recognizing an identity of a person in a video according to the gait thereof through dual-channel convolutional neural networks sharing weights by means of the strong learning capability of the deep learning convolutional neural network. Said method is quite robust to gait changes across a large view, which can effectively solve the problem of low precision in cross-view gait recognition existing with the prior art gait recognition technology. Said method can be widely used in scenarios having video monitors, such as security monitoring in airports and supermarkets, person recognition, criminal detection, etc.
Watrix社を取り上げている記事
複数の媒体が、同社について報じています。
- TechCrunch (2018年11月08日) by Jon Russell 「中国の警察に歩行特徴認識テクノロジー導入――50m離れて後ろ向きでも個人を特定可能」
- Gigazine(2018年11月08日 07時00分) 『「歩き方だけで個人を識別する」監視システムが中国で注目されている』
- BUSINESS INSIDER (2018年11月12日 10:30)「顔を隠しても無駄! 中国の監視カメラは“歩く姿”だけで個人を特定」
- by BRYAN CLARK, China has a new surveillance tool that identifies citizens by how they walk
中国科学院の論文:Finding Tiny Faces in the Wild with Generative Adversarial Network
この連載シリーズでは、中国における(動)画像解析技術の研究開発・運用状況の現状(の水面上に現れた氷山の一角)を、公然情報である公開論文を1つ1つ読み解きながら、見つめて行きたいと思います。
第一弾は、中国科学院(Chinese Academy of Sciences)他から公開された論文を取り上げます。
今日の画像解析器は、画像に写り込んだきわめて小さなサイズの物体についても、画像を鮮明化し、物体識別や人物同定を行う能力を獲得しつつあります。
中国科学院(Chinese Academy of Sciences)他から公開された以下の論文は、人間の群衆の様子を捉えた一枚の画像(wild crowd。論文中では、“wild”と記述)のなかに映り込んでいるすべての人間の表情画像を拡大し、鮮明化させる手法が提案されています。
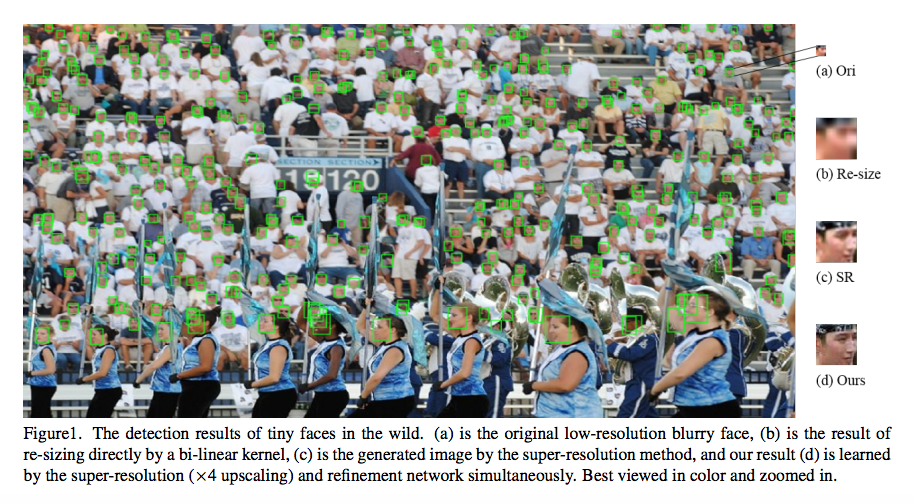
Yancheng Bai, et.al., Finding Tiny Faces in the Wild with Generative Adversarial Networkより転載。
なお、低解像度画像を、高精細化処理器に入力して、高精細な高解像度画像を得る技術としては、「Face Hallucination法」という手法も知られています。
この手法に関しては、すでに取り上げたMegvii社に所属するErjin Zhou氏から、以下の論文が出ています。この論文は2015年度のAAAI誌の査読を通過した論文であり、Erjin Zhou氏の所属先は、当時は北京にある清華大学(Tsinghua University)となっています。なお、清華大学は理系における中国のトップの大学です。
この論文の執筆陣の顔ぶれは、以下の通りです。
- Erjin Zhou氏(Tsinghua University, Beijing, China)
- Haoqiang Fan氏(Tsinghua University, Beijing, China)
- Zhimin Cao氏 (Megvii Technology, Beijing, China)
- Yuning Jiang氏(Megvii Technology, Beijing, China)
- Qi Yin氏(Megvii Technology, Beijing, China)
この論文も、本連載シリーズで見ていくことにします。
では、中国科学院のFinding Tiny Faces in the Wild with Generative Adversarial Network論文に戻ります。
提案手法
GANモデル(敵対的生成モデル)の枠組みを用いることで、極小サイズ且つ低解像度の画像を元に、サイズを大きく拡大し、さらに解像度が高精細化された画像を生成する画像処理モデルを提案しています。
Abstract
Face detection techniques have been developed for decades, and one of remaining open challenges is detecting small faces in unconstrained conditions. The reason is that tiny faces are often lacking detailed information and blurring. In this paper, we proposed an algorithm to directly generate a clear high-resolution face from a blurry small one by adopting a generative adversarial network (GAN). Toward this end, the basic GAN formulation achieves it by super-resolving and refining sequentially (e.g. SR-GAN and cycle-GAN)
提案モデルの入出力関係は、以下の通りです。
入出力関係
- 入力データ:人物の群衆画像データ(10-30pixelサイズの極小の人物表情画像と、看板や背景画像など、Non-faces画像が混在した画像データ。WIDER FACE datasetのHardカテゴリ画像を採用)”the original low-resolution blurry face”
- 出力データ:極小サイズ(10-30pixel)の人物画像を拡大(“up-sampling”)・鮮明化(“refining”)させた人物識別可能な画像 “a clear high-resolution face”
Extensive experiments on the challenging dataset WIDER FACE demonstrate the effectiveness of our proposed method in restoring a clear high-resolution face from a blurry small one, and show that the detection performance outperforms other state-of-the-art methods.
データ処理過程
- 極小画像の拡大処理(“up-sampling”)
- 拡大した画像の鮮明化処理(“refining”):(ぼやけ(“blurry”)・照り(“illumination”)の除去と、非正面の姿勢(“arbitrary poses”)の正面化などを含む処理。
このような、画像の拡大と高精細化(鮮明化)という2段階の処理が施されることで、入力画像(a)は、最終的に(d)の画像に加工されて、モデルから出力されます。
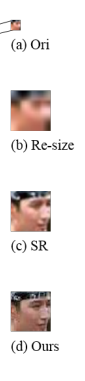
この様子を論文中の別の画像例で説明してみます。
上段(Original)の画像が、提案モデルに入力された元画像です。(但し、実際のサイズは小さすぎて視認しずらいため、下段の拡大された後の画像と同じサイズに修正した上で掲載されている)
そして、下段(Ours)の画像が、対応する上段の画像に拡大処理+鮮明(高精細)化処理が施された後の画像です。この下段の画像が、モデルから出力(生成)される画像です。
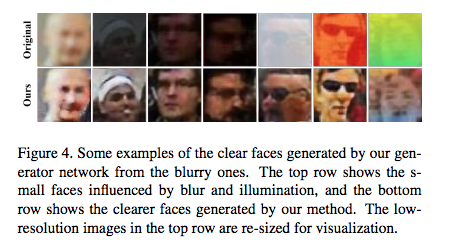
これまでも、画像を高精細化(super-resolution)させるGANは、SR-GANとして提案されてきましたが、生成される画像は、ぼやけた(blurry)画像でした。
提案モデルは、上記の下段の画像を生成できるため、これまでのモデルよりも優れたモデルであるという主張がなされています。
Recently, GAN has been applied to super-resolution (SRGAN) [17] and has obtained promising results.
Compared to superresolution on natural images, face images in the wild are of arbitrary poses, illumination and blur, so super-resolution on face images is much more challenging.
More importantly, the high resolution images generated by SRGAN are blurry and lack fine details especially for low-resolution faces, which are unfriendly for the face classifier
モデルのネットワーク構成
- 極小画像の拡大処理を担う“up-sampling sub-network”
The up-sampling sub-network first up-samples a lowresolution image and outputs a 4× super-resolution image, and this super-resolution image is blurring when the small faces are far from the cameras or under fast motion.
2. 拡大した画像の鮮明化処理を担う“refinement sub-network”
Then, the refinement sub-network processes the blurring image, and outputs a clear super-resolution image, which is easier for the discriminator to classify the faces vs. non-faces.
なお、2.で取り除かれる画像の「ぼやけ」が発生する要因としては、上記の1を記述した文のくだりで、画像を撮影したカメラから遠距離に位置する人物や、高速で移動中(“under fast motion”)の人物を撮影した場合が、例示されています。
this super-resolution image is blurring when the small faces are far from the cameras or under fast motion.
モデル概要:GANモデルを拡張したもの
GAN(敵対的生成モデル:Generative Adversary Network)モデルを拡張したモデルを採用しています。
- GANのGenerator networkのsub network (1): “Up-sample sub-network“:10-30pixelの人物表情画像を画像拡大する(Up-sampling処理)
- GANのGenerator networkのsub network (2): “Refinement sub-network“:拡大した画像から、ぼやけ(blurry)や照り(illumination)を除去し、姿勢を正面を向いた方向に修正する(Refinement処理)
- GANのDiscriminator network:G-subnetwork(2)から出力された生成画像が、(1) 人物の氷像画像であるのか否か(“Face” or “Non-face”) 及び (2) 本物の顔画像か、合成された偽物の顔画像か(“fake” or “real”, HR(the natural (high-resolution) image) or SR “generated super-resolution image”)の2つを判定する。
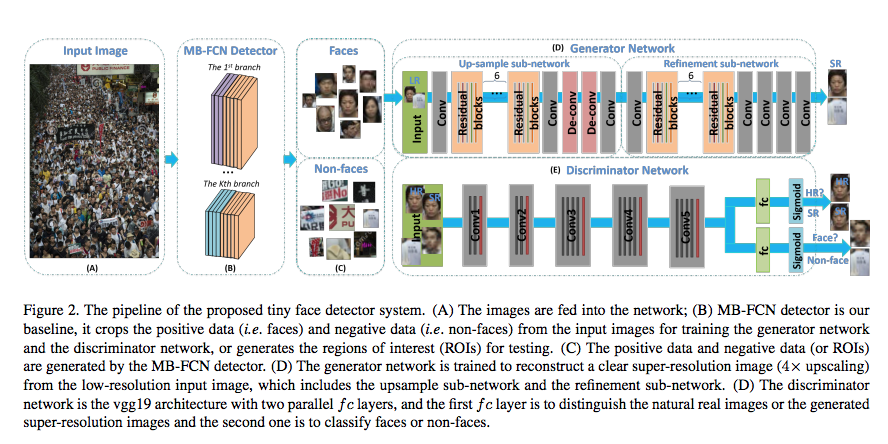
なお、入力データをGANモデルの2本の柱であるGenerator部とDiscriminator部に入力する前に、入力データである群衆画像を構成する個々の部分画像領域が、「人物の顔画像が写り込んだ画像(Faces)」であるのか、「人物の顔画像が映りこんでいない画像(Non-faces)」であるのかを判別する分類器であるMB-FCN Detector が、間に挟み込まれています。
Our baseline MB-FCN detector is based on ResNet50 network [9], which is pre-trained on ImageNet. All hyperparameters of the MB-FCN detector are the same as [1].
For training our generator and discriminator network, we crop face samples and non-face samples from WIDER FACE [31] training set with our baseline detector.
上記の記述にあるように、このMB-FCN Detector(顔画像判定器)は、以下の論文で提案されているモデルを借用しています。
GANモデルの拡張ポイント(3点)
提案モデルは、従来のGANモデルを以下の3点で「拡張」したものです。
(1)Generator部を2つのsub-networkに分けた。(画像の拡張処理部および鮮明化処理部)
(2)Discriminator部を2つの「FC(全結合)層+Sigmoid層」に分けた。(「人物の表情画像か否か」を判定するFC層+Sigmoid層と、「大きく(且つ)鮮明に撮影された画像か、それとも、極小画像を拡大化処理&鮮明化処理を通して得られた画像か」を判定するFC層+Sigmoid層)
(3)損失関数を、3つの損失関数で構成される(1つの)統合損失関数として設計した。
GANモデルの損失関数は、以下の3つの損失関数を最終的に1本の定義式(損失関数式)にまとめたものになります。
3つの損失関数は、(1)Pixel-wise loss. Lmse(w), (2)Adversarial loss. L_{adv} そして (3)Classification loss. L_{clc} です。
- Pixel-wise loss. Lmse(w) : モデルへの入力画像である「小さく、ぼやけた画像」と、モデルが生成した「高精細な画像」を見分ける判別精度を計量計量評価する損失関数。以下の定義式で表現されているように、後者の『モデルが生成した「高精細な画像」』は、まず最初に、I^{LR}_{i}:「小さく、ぼやけた画像」をGenerator1の処理関数(G1_{w1})に投入して画像サイズを拡大させた後に、さらに、Generator2の処理関数(G2_{w2})に投入して画像を鮮明化させた結果として、得られた画像として定義されています。
[期待される役割]
モデルから出力される(拡大処理と鮮明化処理を施された)画像が、「撮影当初から大きくて、鮮明であった画像」(the super-resolution ground truth)に(極限まで)近づくように、モデル全体を制御する役割が期待されています。
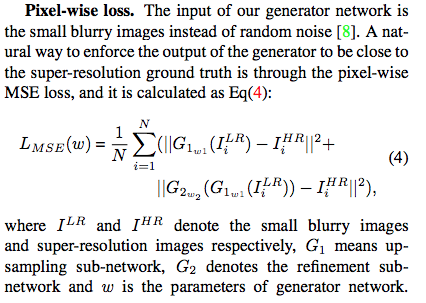
2. Adversarial loss. L_{adv} : 生成された画像が、「撮影当初から高精細(natural super-resolution)であった、本物の画像」であるのか、「撮影当初は不鮮明であった画像を人為的に高精細化処理を施した画像」であるのかの判別精度を計量評価する損失関数
In Eq(5), the Dθ(Gw(I LR i )) is the probability that the reconstruction image Gw(I LR i ) is a natural super-resolution image.
後者の「撮影当初は不鮮明であった画像を人為的に高精細化処理を施した画像」は、「撮影当初は不鮮明であった画像」を表すI^{LR}_{i}(LRは、Low-resolutionを意味する)を、人為的な画像高精細化処理関数であるG_{w}に投入することで得られます。
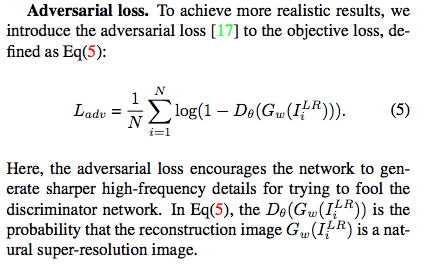
3. Classification loss. L_{clc} : 「ある高精細な画像」(「撮影当初から高精細であった本物の画像」[I^{HR}_{i}: the natural real high resolution images, the high-resolution real natural images)なのか、それとも、「撮影当初は不鮮明であった画像」(I^{LR}_{i}: the small blurry images) に対して、「人為的な高精細化処理」(関数G_{w})を施した「画像」なのか、の別は問わない)が、「人物の顔画像であるか否か」の判別(classify)精度を計量評価する損失関数。
[期待される役割]
提案モデルから最終的に生成される画像が、人物の表情画像か、非ヒト表情画像なのかを判別しやすい画像になるように、モデルに制御をかける役割が期待されている損失関数です。
Our classification loss plays two roles, where the first is to distinguish whether the high-resolution images, including both the generated and the natural real high-resolution images, are faces or non-faces in the discriminator network.
The other role is to promote the generator network to reconstruct sharper images.
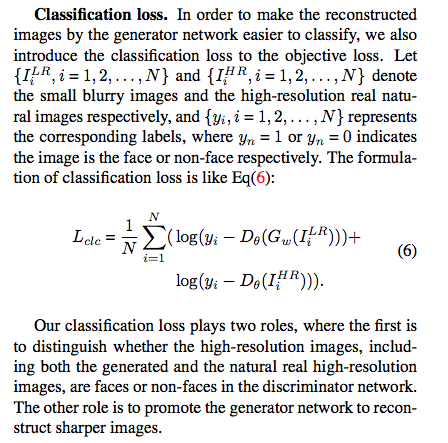
上記の3つの損失関数を1本の損失関数に束ねたものは、以下になります。モデルの学習時に用いられる損失関数は、この損失関数です。
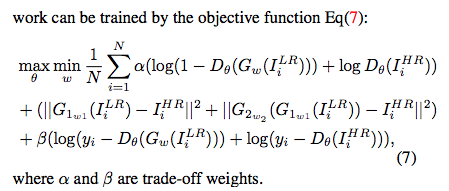
(データセット)
提案モデルの精度を検証するための検証用データセットとして、以下のWIDER FACE dataset中のHardカテゴリのデータセット(画像サイズが10-30pixelの極小サイズのもの)を用いています。
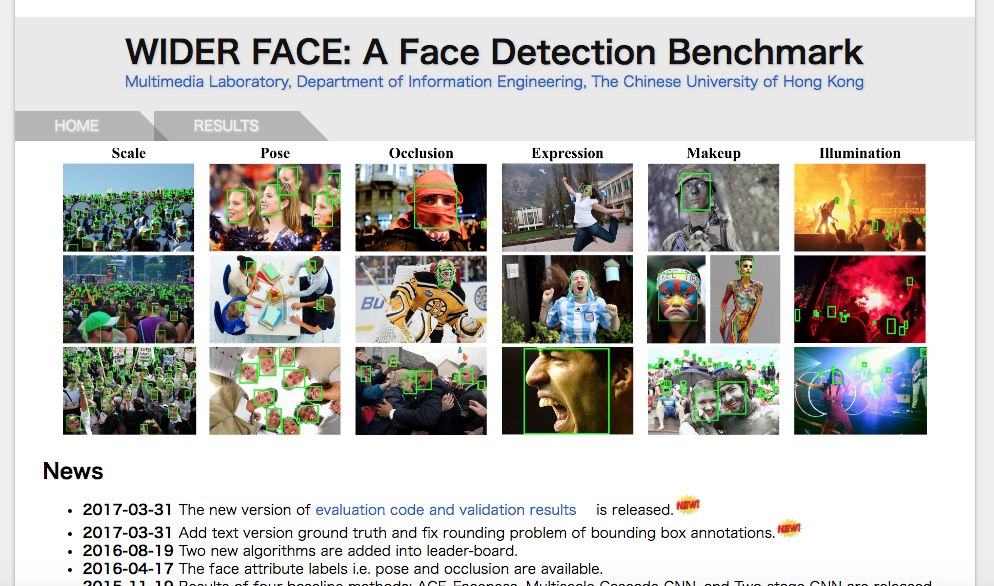
http://mmlab.ie.cuhk.edu.hk/projects/WIDERFace/
4.1. Training and Validation Datasets
We use a recently released large-scale face detection benchmark, the WIDER FACE dataset [31].
It contains 32,203 images, which are selected from the publicly available WIDER dataset.
40%/10%/50% of the data is randomly selected for training, validation, and testing, respectively. Images in WIDER FACE are categorized into 61 social event classes, which have much more diversities and are closer to the real-world scenario.
Therefore, we use this dataset for training and validating the proposed generator and discriminator networks. The WIDER FACE dataset is divided into three subsets, Easy, Medium, and Hard, based on the heights of the ground truth faces.
The Easy/Medium/Hard subsets contain faces with heights larger than 50/30/10 pixels respectively. Compared to the Medium subset, the Hard one contains many faces with a height between 10−30 pixels.
As expected, it is quite challenging to achieve good detection performance on the Hard subset.
(同じ執筆陣による同様の論文)
なお、同じ執筆陣による似た内容の論文としては、以下があります。こちらの論文は、画像認識分野で世界最高峰の学会誌であるECCV(European Conference on Computer Vision)の査読を通過し、掲載(2018年度)されたもので、”MTGAN”モデルを提案しています。(”an end-to-end multi-task generative adversarial network“)
Abstract.
Object detection is a fundamental and important problem in computer vision.
Although impressive results have been achieved on large/medium sized objects in large-scale detection benchmarks (e.g. the COCO dataset), the performance on small objects is far from satisfactory.
The reason is that small objects lack sufficient detailed appearance information, which can distinguish them from the background or similar objects.
To deal with the small object detection problem, we propose an end-to-end multi-task generative adversarial network (MTGAN).
In the MTGAN, the generator is a super-resolution network, which can up-sample small blurred images into fine-scale ones and recover detailed information for more accurate detection.
The discriminator is a multitask network, which describes each super-resolved image patch with a real/fake score, object category scores, and bounding box regression offsets.
Furthermore, to make the generator recover more details for easier detection, the classification and regression losses in the discriminator are back-propagated into the generator during training.
Extensive experiments on the challenging COCO dataset demonstrate the effectiveness of the proposed method in restoring a clear super-resolved image from a blurred small one, and show that the detection performance, especially for small sized objects, improves over state-of-the-art methods.
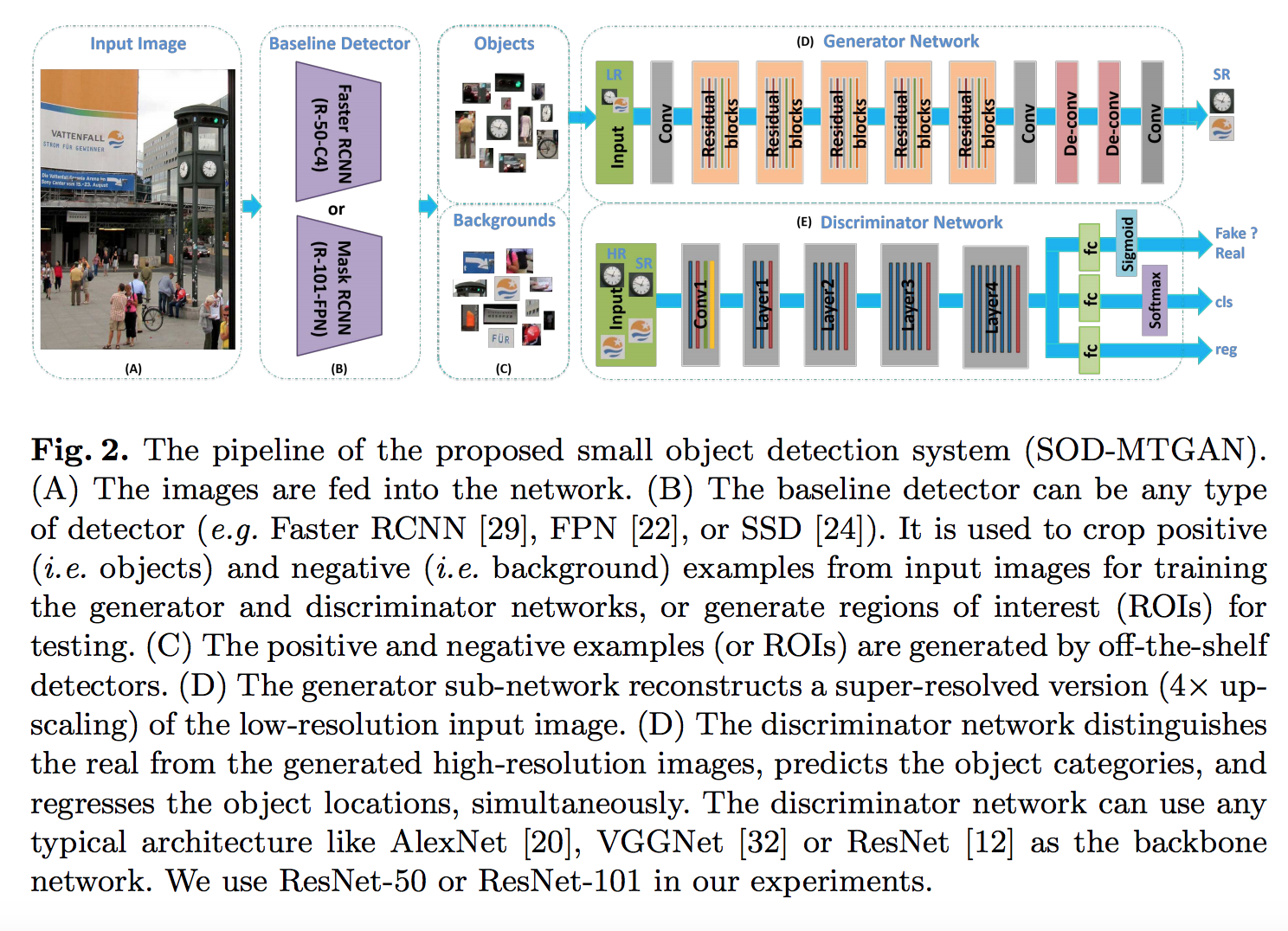
Yancheng Bai et.al., SOD-MTGAN: Small Object Detection via Multi-Task Generative Adversarial Network, ECCV’18より抜粋。
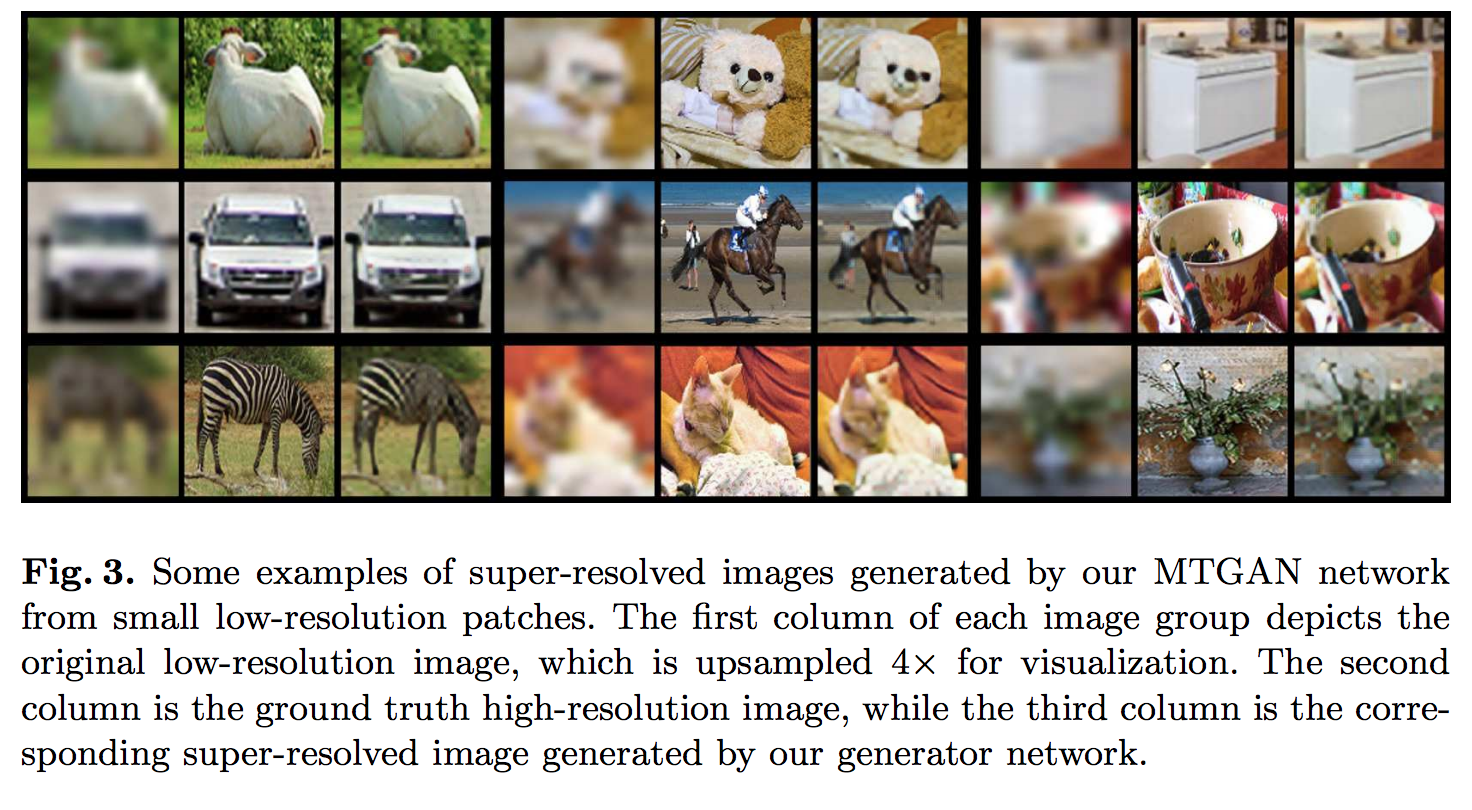
Yancheng Bai et.al., SOD-MTGAN: Small Object Detection via Multi-Task Generative Adversarial Network, ECCV’18より抜粋。
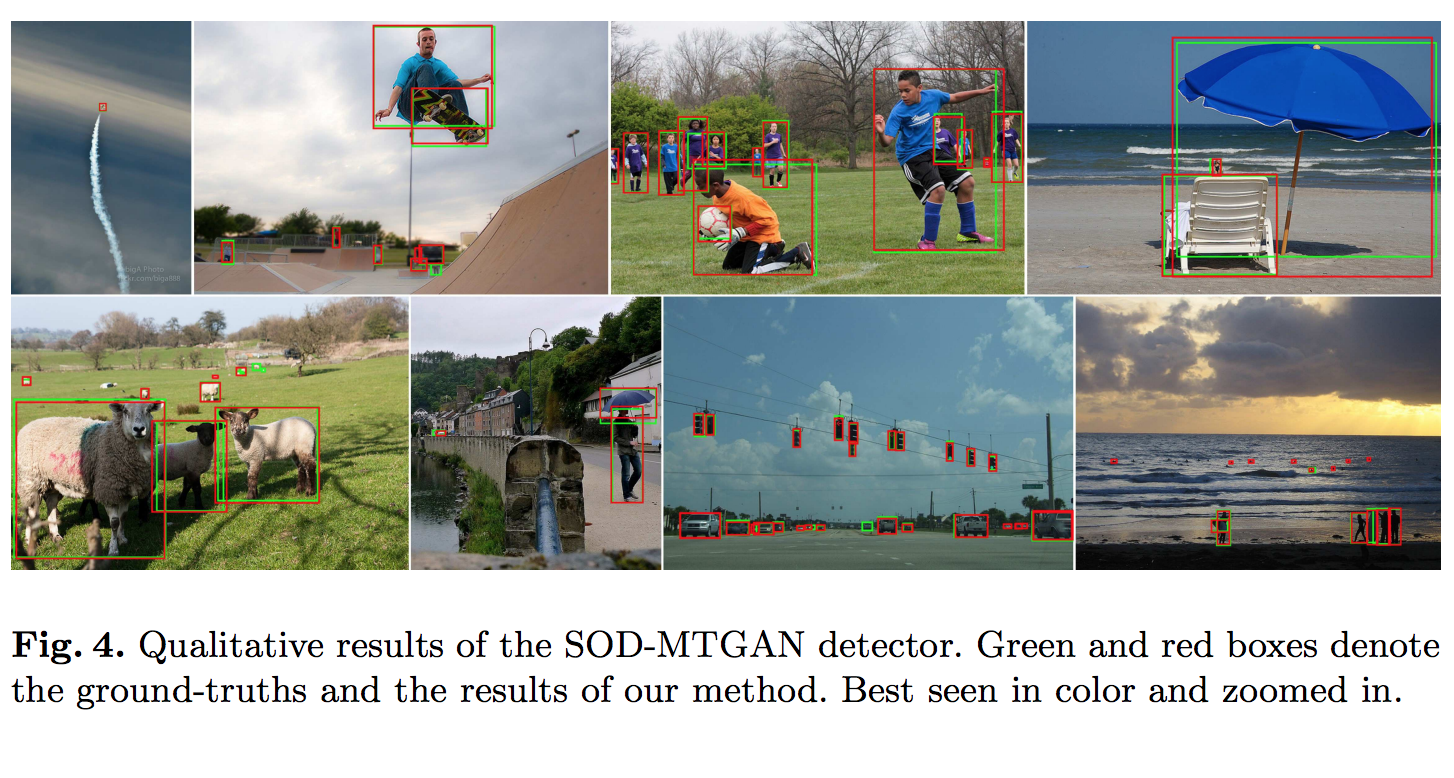
Yancheng Bai et.al., SOD-MTGAN: Small Object Detection via Multi-Task Generative Adversarial Network, ECCV’18より抜粋。
(関連論文)
ぼやけた(blurring)画像や、他の物体の物陰と重なるなどして見えにくくなった物体の画像(occluded)を鮮明化・高解像度化する技術を提案している論文としては、例えば以下があります。
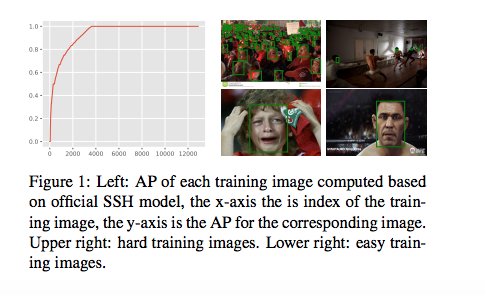
Zhishuai Zhang et.al., Robust Face Detection via Learning Small Faces on Hard Images, Arxiv 2018より転載。